The Rise of AI
Artificial Intelligence (AI) is a broad discipline focused on creating machines capable of mimicking human intelligence and more specifically…learning. It even dates back to the 1950s.
These tasks might include understanding natural language, recognizing images, solving complex problems, and even driving cars. Unlike traditional software, AI systems can learn from experience, adapt to new inputs, and perform human-like tasks by processing large amounts of data.
Today, around 42% of companies have reported exploring AI use within their company, and over 50% of companies plan to incorporate AI technologies in 2024. The AI Market is expected to reach a staggering $407 billion by 2027.
What Is the Difference Between AI, ML and LLM?
AI encompasses a vast range of technologies, including Machine Learning (ML), Generative AI (GAI), and Large Language Models (LLM), among others.
Machine Learning, a subset of AI, was developed in the 1980s. Its main focus is on enabling machines to learn from data, improve their performance, and make decisions without explicit programming. Google's search algorithm is a prime example of an ML application, using previous data to refine search results.
Generative AI (GAI), evolved from ML in the early 21st century, represents a class of algorithms capable of generating new data. They construct data that resembles the input, making them essential in fields like content creation and data augmentation.
Large Language Models (LLM) also arose from the GAI subset. LLMs generate human-like text by predicting the likelihood of a word given the previous words used in the text. They are the core technology behind many voice assistants and chatbots. One of the most well-known examples of LLMs is OpenAI's ChatGPT model.
LLMs are trained on huge sets of data — which is why they are called "large" language models. LLMs are built on machine learning: specifically, a type of neural network called a transformer model.
In simpler terms, an LLM is a computer program that has been fed enough examples to be able to recognize and interpret human language or other types of complex data. Many LLMs are trained on data that has been gathered from the Internet — thousands or even millions of gigabytes' worth of text. But the quality of the samples impacts how well LLMs will learn natural language, so LLM's programmers may use a more curated data set.
Here are some of the main functions LLMs currently serve:
- Natural language generation
- Language translation
- Sentiment analysis
- Content creation
What is AI SPM?
AI-SPM (artificial intelligence security posture management) is a comprehensive approach to securing artificial intelligence and machine learning. It includes identifying and addressing vulnerabilities, misconfigurations, and potential risks associated with AI applications and training data sets, as well as ensuring compliance with relevant data privacy and security regulations.
How Can AI Help Data Security?
With data breaches and cyber threats becoming increasingly sophisticated, having a way of securing data with AI is paramount. AI-powered security systems can rapidly identify and respond to potential threats, learning and adapting to new attack patterns faster than traditional methods. According to a 2023 report by IBM, the average time to identify and contain a data breach was reduced by nearly 50% when AI and automation were involved.
By leveraging machine learning algorithms, these systems can detect anomalies in real-time, ensuring that sensitive information remains protected. Furthermore, AI can automate routine security tasks, freeing up human experts to focus on more complex challenges. Ultimately, AI-driven data security not only enhances protection but also provides a robust defense against evolving cyber threats, safeguarding both personal and organizational data.
What Do You Need to Secure
So now that we have defined Artificial Intelligence, Machine Learning and Large Language Models, it’s time to get familiar with the data flow and its components. Understanding the data flows can help us identify those vulnerable points where we can improve data security.
The process can be illustrated with the following flow:
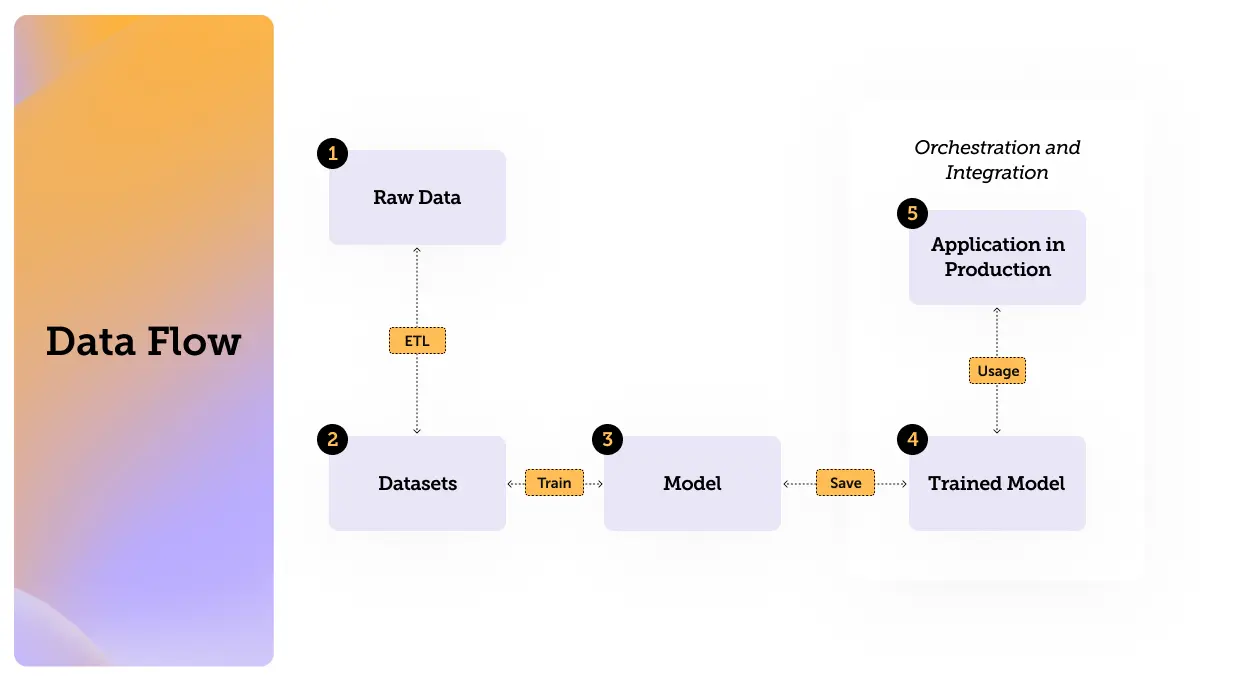
(If you are already familiar with datasets models and everything in between feel free to jump straight to the threats section)
Understanding Training Datasets
The main component of the first stage we will discuss is the training dataset.
Training datasets are collections of labeled or unlabeled data used to train, validate, and test machine learning models. They can be identified by their structured nature and the presence of input-output pairs for supervised learning.
Training datasets are essential for training models, as they provide the necessary information for the model to learn and make predictions. They can be manually created, parsed using tools like Glue and ETLs, or sourced from predefined open-source datasets such as those from HuggingFace, Kaggle, and GitHub.
Training datasets can be stored locally on personal computers, virtual servers, or in cloud storage services such as AWS S3, RDS, and Glue.
Examples of training datasets include image datasets for computer vision tasks, text datasets for natural language processing, and tabular datasets for predictive modeling.
What is a Machine Learning Model?
This brings us to the next component: models.
A model in machine learning is a mathematical representation that learns from data to make predictions or decisions. Models can be pre-trained, like GPT-4, GPT-4.5, and LLAMA, or developed in-house.
Models are trained using training datasets. The training process involves feeding the model data so it can learn patterns and relationships within the data. This process requires compute power and be done using containers, or services such as AWS SageMaker and Bedrock. The output is a bunch of parameters that are used to fine tune the model. If someone gets their hand on those parameters it's as if they trained the model themselves.
Once trained, models can be used to predict outcomes based on new inputs. They are deployed in production environments to perform tasks such as classification, regression, and more.
How Data Flows: Orchestration and Integration
This leads us to our last stage which is the Orchestration and Integration (Flow).
These tools manage the deployment and execution of models, ensuring they perform as expected in production environments. They handle the workflow of machine learning processes, from data ingestion to model deployment.
Integration: Integrating models into applications involves using APIs and other interfaces to allow seamless communication between the model and the application. This ensures that the model's predictions are utilized effectively.
Possible Threats: Orchestration tools can be exploited to perform LLM attacks, where vulnerabilities in the deployment and management processes are targeted.
We will cover this in the next chapter of this article.
Conclusion
We reviewed what AI is composed of and examined the individual components, including data flows and how they function within the broader AI ecosystem. In the part 2 episode of this 3 part series, we’ll explore LLM attack techniques and threats.
With Sentra, your team will gain visibility and control into any training dataset, models and AI applications in your cloud environments, such as AWS. By using Sentra, you can minimize data security risks in our AI applications and ensure they remain secure without sacrificing efficiency or performance. Sentra can help you navigate the complexities of AI security, providing the tools and knowledge necessary to protect your data and maximize the potential of your AI initiatives.