How Sentra Built a Data Security Platform for the AI Era
In just three years, Sentra has witnessed the rapid evolution of the data security landscape. What began with traditional on-premise Data Loss Prevention (DLP) solutions has shifted to a cloud-native focus with Data Security Posture Management (DSPM). This marked a major leap in how organizations protect their data, but the evolution didn’t stop there.
The next wave introduced new capabilities like Data Detection and Response (DDR) and Data Access Governance (DAG), pushing the boundaries of what DSPM could offer. Now, we’re entering an era where SaaS Security Posture Management (SSPM) and Artificial Intelligence Security Posture Management (AI-SPM) are becoming increasingly important.
These shifts are redefining what we’ve traditionally called Data Security Platform (DSP) solutions, marking a significant transformation in the industry. The speed of this evolution speaks to the growing complexity of data security needs and the innovation required to meet them.
The Evolution of Data Security

What Is Driving The Evolution of Data Security?
The evolution of the data security market is being driven by several key macro trends:
- Digital Transformation and Data Democratization: Organizations are increasingly embracing digital transformation, making data more accessible to various teams and users.
- Rapid Cloud Adoption: Businesses are moving to the cloud at an unprecedented pace to enhance agility and responsiveness.
- Explosion of Siloed Data Stores: The growing number of siloed data stores, diverse data technologies, and an expanding user base is complicating data management.
- Increased Innovation Pace: The rise of artificial intelligence (AI) is accelerating the pace of innovation, creating new opportunities and challenges in data security.
- Resource Shortages: As organizations grow, the need for automation to keep up with increasing demands has never been more critical.
- Stricter Data Privacy Regulations: Heightened data privacy laws and stricter breach disclosure requirements are adding to the urgency for robust data protection measures.
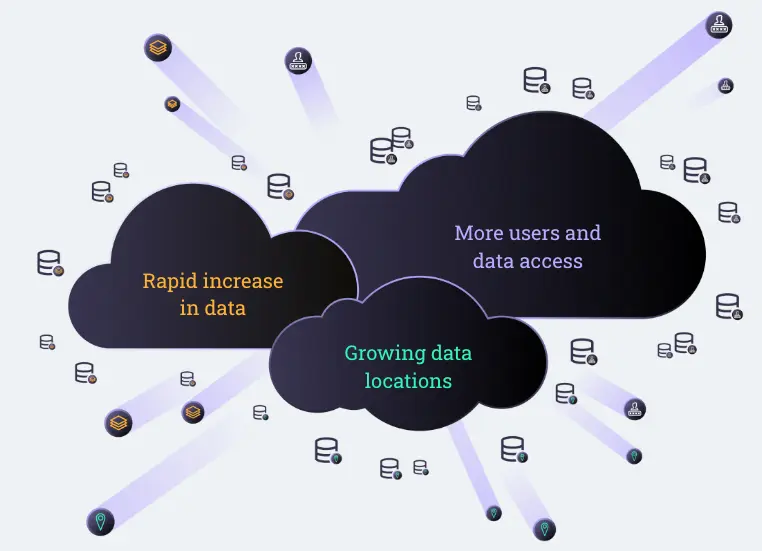
Similarly, there has been an evolution in the roles involved with the management, governance, and protection of data. These roles are increasingly intertwined and co-dependent as described in our recent blog entitled “Data: The Unifying Force Behind Disparate GRC Functions”. We identify that today each respective function operates within its own domain yet shares ownership of data at its core. As the co-dependency on data increases so does the need for a unifying platform approach to data security.
Sentra has adapted to these changes to align our messaging with industry expectations, buyer requirements, and product/technology advancements.
A Data Security Platform for the AI Era
Sentra is setting the standard with the leading Data Security Platform for the AI Era.
With its cloud-native design, Sentra seamlessly integrates powerful capabilities like Data Discovery and Classification, Data Security Posture Management (DSPM), Data Access Governance (DAG), and Data Detection and Response (DDR) into a comprehensive solution. This allows our customers to achieve enterprise-scale data protection while addressing critical questions about their data.

What sets Sentra apart is its connector-less, cloud-native architecture, which effortlessly scales to accommodate multi-petabyte, multi-cloud environments without the administrative burdens typical of connector-based legacy systems. These more labor-intensive approaches often struggle to keep pace and frequently overlook shadow data.
Moreover, Sentra harnesses the power of AI and machine learning to accurately interpret data context and classify data. This not only enhances data security but also ensures the privacy and integrity of data used in Gen- AI applications. We recognized the critical need for accurate and automated Data Discovery and Classification, along with Data Security Posture Management (DSPM), to address the risks associated with data proliferation in a multi-cloud landscape. Based on our customers' evolving needs, we expanded our capabilities to include DAG and DDR. These tools are essential for managing data access, detecting emerging threats, and improving risk mitigation and data loss prevention.
DAG maps the relationships between cloud identities, roles, permissions, data stores, and sensitive data classes. This provides a complete view of which identities and data stores in the cloud may be overprivileged. Meanwhile, DDR offers continuous threat monitoring for suspicious data access activity, providing early warnings of potential breaches.
We grew to support SaaS data repositories including Microsoft 365 (SharePoint, OneDrive, Teams, etc.), G Suite (Gdrive) and leveraged AI/ML to accurately classify data hidden within unstructured data stores.
Sentra’s accurate data sensitivity tagging and granular contextual details allows organizations to enhance the effectiveness of their existing tools, streamline workflows, and automate remediation processes. Additionally, Sentra offers pre-built integrations with various analysis and response tools used across the enterprise, including data catalogs, incident response (IR) platforms, IT service management (ITSM) systems, DLPs, CSPMs, CNAPPs, IAM, and compliance management solutions.
How Sentra Redefines Enterprise Data Security Across Clouds
Sentra has architected a solution that can deliver enterprise-scale data security without the traditional constraints and administrative headaches. Sentra’s cloud-native design easily scales to petabyte data volumes across multi-cloud and on-premises environments.
The Sentra platform incorporates a few major differentiators that distinguish it from other solutions including:
- Novel Scanning Technology: Sentra uses inventory files and advanced automatic grouping to create a new entity called “Data Asset”, a group of files that have the same structure, security posture and business function. Sentra automatically reduces billions of files into thousands of data assets (that represent different types of data) continuously, enabling full coverage of 100% of cloud data of petabytes to just several hundreds of thousands of files which need to be scanned (5-6 orders of magnitude less scanning required). Since there is no random sampling involved in the process, all types of data are fully scanned and for differentials on a daily basis. Sentra supports all leading IaaS, PaaS, SaaS and On-premises stores.
- AI-powered Autonomous Classification: Sentra’s use of AI-powered classification provides approximately 97% classification accuracy of data within unstructured documents and structured data. Additionally, Sentra provides rich data context (distinct from data class or type) about multiple aspects of files, such as data subject residency, business impact, synthetic or real data, and more. Further, Sentra’s classification uses LLMs (inside the customer environment) to automatically learn and adapt based on the unique business context, false positive user inputs, and allows users to add AI-based classifiers using natural language (powered by LLMs). This autonomous learning means users don’t have to customize the system themselves, saving time and helping to keep pace with dynamic data.
- Data Perimeters / Movement: Sentra DataTreks™ provides the ability to understand data perimeters automatically and detect when data is moving (e.g. copied partially or fully) to a different perimeter. For example, it can detect data similarity/movement from a well protected production environment to a less- protected development environment. This is important for highly dynamic cloud environments and promoting secure data democratization.
- Data Detection and Response (DDR): Sentra’s DDR module highlights anomalies such as unauthorized data access or unusual data movements in near real-time, integrating alerts into existing tools like ServiceNow or JIRA for quick mitigation.
- Easy Customization: In addition to ‘learning’ of a customer's unique data types, with Sentra it’s easy to create new classifiers, modify policies, and apply custom tagging labels.
As AI reshapes the digital landscape, it also creates new vulnerabilities, such as the risk of data exposure through AI training processes. The Sentra platform addresses these AI-specific challenges, while continuing to tackle the persistent security issues from the cloud era, providing an integrated solution that ensures data security remains resilient and adaptive.
Use Cases: Solving Complex Problems with Unique Solutions
Sentra’s unique capabilities allow it to serve a broad spectrum of challenging data security, governance and compliance use cases. Two frequently cited DSPM use cases are preventing data breaches and facilitating GenAI technology deployments. With the addition of data privacy compliance, these represent the top three.
Let's dive deeper into how Sentra's platform addresses specific challenges:
Data Risk Visibility
Sentra’s Data Security Platform enables continuous analysis of your security posture and automates risk assessments across your entire data landscape. It identifies data vulnerabilities across cloud-native and unmanaged databases, data lakes, and metadata catalogs. By automating the discovery and classification of sensitive data, teams can prioritize actions based on the sensitivity and policy guidelines related to each asset. This automation not only saves time but also enhances accuracy, especially when leveraging large language models (LLMs) for detailed data classification.
Security and Compliance Audit
Sentra Data Security Platform can also automate the process of identifying regulatory violations and ensuring adherence to custom and pre-built policies (including policies that map to common compliance frameworks).
The platform automates the identification of regulatory violations, ensuring compliance with both custom and established policies. It helps keep sensitive data in the right environments, preventing it from traveling to regions that violate retention policies or lack encryption. Unlike manual policy implementation, which is prone to errors, Sentra’s automated approach significantly reduces the risk of misconfiguration, ensuring that teams don’t miss critical activities.
Data Access Governance
Sentra enhances data access governance (DAG) by enforcing appropriate permissions for all users and applications within an organization. By automating the monitoring of access permissions, Sentra mitigates risks such as excessive permissions and unauthorized access. This ensures that teams can maintain least privilege access control, which is essential in a growing data ecosystem.
Minimizing Data and Attack Surface
The platform’s capabilities also extend to detecting unmanaged sensitive data, such as shadow or duplicate assets. By automatically finding and classifying these unknown data points, Sentra minimizes the attack surface, controls data sprawl, and enhances overall data protection.
Secure and Responsible AI
As organizations build new Generative AI applications, Sentra extends its protection to LLM applications, treating them as part of the data attack surface. This proactive management, alongside monitoring of prompts and outputs, addresses data privacy and integrity concerns, ensuring that organizations are prepared for the future of AI technologies.
Insider Risk Management
Sentra effectively detects insider risks by monitoring user access to sensitive information across various platforms. Its Data Detection and Response (DDR) capabilities provide real-time threat detection, analyzing user activity and audit logs to identify unusual patterns.
Data Loss Prevention (DLP)
The platform integrates seamlessly with endpoint DLP solutions to monitor all access activities related to sensitive data. By detecting unauthorized access attempts from external networks, Sentra can prevent data breaches before they escalate, all while maintaining a positive user experience.
Sentra’s robust Data Security Platform offers solutions for these use cases and more, empowering organizations to navigate the complexities of data security with confidence. With a comprehensive approach that combines visibility, governance, and protection, Sentra helps businesses secure their data effectively in today’s dynamic digital environment.
From DSPM to a Comprehensive Data Security Platform
Sentra has evolved beyond being the leading Data Security Posture Management (DSPM) solution; we are now a Cloud-native Data Security Platform (DSP). Today, we offer holistic solutions that empower organizations to locate, secure, and monitor their data against emerging threats. Our mission is to help businesses move faster and thrive in today’s digital landscape.
What sets the Sentra DSP apart is its unique layer of protection, distinct from traditional infrastructure-dependent solutions. It enables organizations to scale their data protection across ever-expanding multi-cloud environments, meeting enterprise demands while adapting to ever-changing business needs—all without placing undue burdens on the teams managing it.
And we continue to progress. In a world rapidly evolving with advancements in AI, the Sentra Data Security Platform stands as the most comprehensive and effective solution to keep pace with the challenges of the AI age. We are committed to developing our platform to ensure that your data security remains robust and adaptive.
