Ron Reiter
Discover Ron’s expertise, shaped by over 20 years of hands-on tech and leadership experience in cybersecurity, cloud, big data, and machine learning. As a serial entrepreneur and seed investor, Ron has contributed to the success of several startups, including Axonius, Firefly, Guardio, Talon Cyber Security, and Lightricks, after founding a company acquired by Oracle.
Name's Data Security Posts
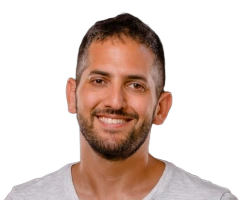
AI in Data Security: Guardian Angel or Trojan Horse?
AI in Data Security: Guardian Angel or Trojan Horse?
Artificial intelligence (AI) is transforming industries, empowering companies to achieve greater efficiency, and maintain a competitive edge. But here’s the catch: although AI unlocks unprecedented opportunities, its rapid adoption also introduces complex challenges—especially for data security and privacy.
How do you accelerate transformation without compromising the integrity of your data? How do you harness AI’s power without it becoming a threat?
For security leaders, AI presents this very paradox. It is a powerful tool for mitigating risk through better detection of sensitive data, more accurate classification, and real-time response. However, it also introduces complex new risks, including expanded attack surfaces, sophisticated threat vectors, and compliance challenges.
As AI becomes ubiquitous and enterprise data systems become increasingly distributed, organizations must navigate the complexities of the big-data AI era to scale AI adoption safely.
In this article, we explore the emerging challenges of using AI in data security and offer practical strategies to help organizations secure sensitive data.
The Emerging Challenges for Data Security with AI
AI-driven systems are driven by vast amounts of data, but this reliance introduces significant security risks—both from internal AI usage and external client-side AI applications. As organizations integrate AI deeper into their operations, security leaders must recognize and mitigate the growing vulnerabilities that come with it.
Below, we outline the four biggest AI security challenges that will shape how you protect data and how you can address them.
1. Expanded Attack Surfaces
AI’s dependence on massive datasets—often unstructured and spread across cloud environments—creates an expansive attack surface. This data sprawl increases exposure to adversarial threats, such as model inversion attacks, where bad actors can reverse-engineer AI models to extract sensitive attributes or even re-identify anonymized data.
To put this in perspective, an AI system trained on healthcare data could inadvertently leak protected health information (PHI) if improperly secured. As adversaries refine their techniques, protecting AI models from data leakage must be a top priority.
For a detailed analysis of this challenge, refer to NIST’s report, “Adversarial Machine Learning: A Taxonomy and Terminology of Attacks and Mitigations.”
2. Sophisticated and Evolving Threat Landscape
The same AI advancements that enable organizations to improve detection and response are also empowering threat actors. Attackers are leveraging AI to automate and enhance malicious campaigns, from highly targeted phishing attacks to AI-generated malware and deepfake fraud.
According to StrongDM's “The State of AI in Cybersecurity Report,” 65% of security professionals believe their organizations are unprepared for AI-driven threats. This highlights a critical gap: while AI-powered defenses continue to improve, attackers are innovating just as fast—if not faster. Organizations must adopt AI-driven security tools and proactive defense strategies to keep pace with this rapidly evolving threat landscape.
3. Data Privacy and Compliance Risks
AI’s reliance on large datasets introduces compliance risks for organizations bound by regulations such as GDPR, CCPA, or HIPAA. Improper handling of sensitive data within AI models can lead to regulatory violations, fines, and reputational damage. One of the biggest challenges is AI’s opacity—in many cases, organizations lack full visibility into how AI systems process, store, and generate insights from data. This makes it difficult to prove compliance, implement effective governance, or ensure that AI applications don’t inadvertently expose personally identifiable information (PII). As regulatory scrutiny on AI increases, businesses must prioritize AI-specific security policies and governance frameworks to mitigate legal and compliance risks.
4. Risk of Unintentional Data Exposure
Even without malicious intent, generative AI models can unintentionally leak sensitive or proprietary data. For instance, employees using AI tools may unknowingly input confidential information into public models, which could then become part of the model’s training data and later be disclosed through the model’s outputs. Generative AI models—especially large language models (LLMs)—are particularly susceptible to data extrapolation attacks, where adversaries manipulate prompts to extract hidden information.
Techniques like “divergence attacks” on ChatGPT can expose training data, including sensitive enterprise knowledge or personally identifiable information. The risks are real, and the pace of AI adoption makes data security awareness across the organization more critical than ever.
For further insights, explore our analysis of “Emerging Data Security Challenges in the LLM Era.”
Top 5 Strategies for Securing Your Data with AI
To integrate AI responsibly into your security posture, companies today need a proactive approach is essential. Below we outline five key strategies to maximize AI’s benefits while mitigating the risks posed by evolving threats. When implemented holistically, these strategies will empower you to leverage AI’s full potential while keeping your data secure.
1. Data Minimization, Masking, and Encryption
The most effective way to reduce risk exposure is by minimizing sensitive data usage whenever possible. Avoid storing or processing sensitive data unless absolutely necessary. Instead, use techniques like synthetic data generation and anonymization to replace sensitive values during AI training and analysis.
When sensitive data must be retained, data masking techniques—such as name substitution or data shuffling—help protect confidentiality while preserving data utility. However, if data must remain intact, end-to-end encryption is critical. Encrypt data both in transit and at rest, especially in cloud or third-party environments, to prevent unauthorized access.
2. Data Governance and Compliance with AI-SPM
Governance and compliance frameworks must evolve to account for AI-driven data processing. AI Security Posture Management (AI-SPM) tools help automate compliance monitoring and enforce governance policies across hybrid and cloud environments.
AI-SPM tools enable:
- Automated data lineage mapping to track how sensitive data flows through AI systems.
- Proactive compliance monitoring to flag data access violations and regulatory risks before they become liabilities.
By integrating AI-SPM into your security program, you ensure that AI-powered workflows remain compliant, transparent, and properly governed throughout their lifecycle.
3. Secure Use of AI Cloud Tools
AI cloud tools accelerate AI adoption, but they also introduce unique security risks. Whether you’re developing custom models or leveraging pre-trained APIs, choosing trusted providers like Amazon Bedrock or Google’s Vertex AI ensures built-in security protections.
However, third-party security is not a substitute for internal controls. To safeguard sensitive workloads, your organization should:
- Implement strict encryption policies for all AI cloud interactions.
- Enforce data isolation to prevent unauthorized access.
- Regularly review vendor agreements and security guarantees to ensure compliance with internal policies.
Cloud AI tools can enhance your security posture, but always review the guarantees of your AI providers (e.g., OpenAI's security and privacy page) and regularly review vendor agreements to ensure alignment with your company’s security policies.
4. Risk Assessments and Red Team Testing
While offline assessments provide an initial security check, AI models behave differently in live environments—introducing unpredictable risks. Continuous risk assessments are critical for detecting vulnerabilities, including adversarial threats and data leakage risks.
Additionally, red team exercises simulate real-world AI attacks before threat actors can exploit weaknesses. A proactive testing cycle ensures AI models remain resilient against emerging threats.
To maintain AI security over time, adopt a continuous feedback loop—incorporating lessons learned from each assessment to strengthen your AI systems
5. Organization-Wide AI Usage Guidelines
AI security isn’t just a technical challenge—it’s an organizational imperative. To democratize AI security, companies must embed AI risk awareness across all teams.
- Establish clear AI usage policies based on zero trust and least privilege principles.
- Define strict guidelines for data sharing with AI platforms to prevent shadow AI risks.
- Integrate AI security into broader cybersecurity training to educate employees on emerging AI threats.
By fostering a security-first culture, organizations can mitigate AI risks at scale and ensure that security teams, developers, and business leaders align on responsible AI practices.
Key Takeaways: Moving Towards Proactive AI Security
AI is transforming how we manage and protect data, but it also introduces new risks that demand ongoing vigilance. By taking a proactive, security-first approach, you can stay ahead of AI-driven threats and build a resilient, future-ready AI security framework.
AI integration is no longer optional for modern enterprises—it is both inevitable and transformative. While AI offers immense potential, particularly in security applications, it also introduces significant risks, especially around data security. Organizations that fail to address these challenges proactively risk increased exposure to evolving threats, compliance failures, and operational disruptions.
By implementing strategies such as data minimization, strong governance, and secure AI adoption, organizations can mitigate these risks while leveraging AI’s full potential. A proactive security approach ensures that AI enhances—not compromises—your overall cybersecurity posture. As AI-driven threats evolve, investing in comprehensive, AI-aware security measures is not just a best practice but a competitive necessity. Sentra’s Data Security Platform provides the necessary visibility and control, integrating advanced AI security capabilities to protect sensitive data across distributed environments.
To learn how Sentra can strengthen your organization’s AI security posture with continuous discovery, automated classification, threat monitoring, and real-time remediation, request a demo today.
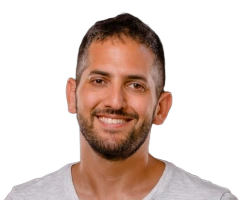
Enhancing AI Governance: The Crucial Role of Data Security
Enhancing AI Governance: The Crucial Role of Data Security
In today’s hyper-connected world, where big data powers decision-making, artificial intelligence (AI) is transforming industries and user experiences around the globe. Yet, while AI technology brings exciting possibilities, it also raises pressing concerns, particularly related to security, compliance, and ethical integrity.
As AI adoption accelerates一fueled by increasingly vast and unstructured data sources—organizations seeking to secure AI deployments (and investments) must establish a strong AI governance initiative with data governance at its core.
This article delves into the essentials of AI governance, outlines its importance, examines the challenges involved, and presents best practices to help companies implement a resilient, secure, and ethically sound AI governance framework centered around data.
What is AI Governance?
AI governance encompasses the frameworks, practices, and policies that guide the responsible, safe, and ethical use of AI systems across an organization. Effective AI governance integrates technical elements—data, models, and code—with human oversight for a holistic framework that evolves alongside an organization’s AI initiatives.
Embedding AI governance, along with related data security measures, into organizational practices not only guarantees responsible AI use but also long-term success in an increasingly AI-driven world.
With an AI governance structure rooted in secure data practices, your company can:
- Mitigate risks: Ongoing AI risk assessments can proactively identify and address potential threats, such as algorithmic bias, transparency gaps, and potential data leakage; this ensures fairer AI outcomes while minimizing reputational and regulatory risks tied to flawed or opaque AI systems.
- Ensure strict adherence: Effective AI governance and compliance policies create clear accountability structures, aligning AI deployments and data use with both internal guidelines and the broader regulatory landscape such as data privacy laws or industry-specific AI standards.
- Optimize AI performance: Centralized AI governance provides full visibility into your end-to-end AI deployments一from data sources and engineered feature sets to trained models and inference endpoints; this facilitates faster and more reliable AI innovations while reducing security vulnerabilities.
- Foster trust: Ethical AI governance practices, backed by strict data security, reinforce trust by ensuring AI systems are transparent and safe, which is crucial for building confidence among both internal and external stakeholders.
A robust AI governance framework means your organization can safeguard sensitive data, build trust, and responsibly harness AI’s transformative potential, all while maintaining a transparent and aligned approach to AI.
Why Data Governance Is at the Center of AI Governance
Data governance is key to effective AI governance because AI systems require high-quality, secure data to properly function. Accurate, complete, and consistent data is a must for AI performance and the decisions that guide it. Additionally, strong data governance enables organizations to navigate complex regulatory landscapes and mitigate ethical concerns related to bias.
Through a structured data governance framework, organizations can not only achieve compliance but also leverage data as a strategic asset, ultimately leading to more reliable and ethical AI outcomes.
Risks of Not Having a Data-Driven AI Governance Framework
AI systems are inherently complex, non-deterministic, and highly adaptive—characteristics that pose unique challenges for governance.
Many organizations face difficulty blending AI governance with their existing data governance and IT protocols; however, a centralized approach to governance is necessary for comprehensive oversight. Without a data-centric AI governance framework, organizations face risks such as:
- Opaque decision-making: Without clear lineage and governance, it becomes difficult to trace and interpret AI decisions, which can lead to unethical, discriminatory, or harmful outcomes.
- Data breaches: AI systems rely on large volumes of data, making rigorous data security protocols essential to avoid leaks of sensitive information across an extended attack surface covering both model inputs and outputs.
- Regulatory non-compliance: The fast-paced evolution of AI regulations means organizations without a governance framework risk large penalties for non-compliance and potential reputational damage.
For more insights on managing AI and data privacy compliance, see our tips for security leaders.
Implementing AI Governance: A Balancing Act
While centralized, robust AI governance is crucial, implementing it successfully poses significant challenges. Organizations must find a balance between driving innovation and maintaining strict oversight of AI operations.
A primary issue is ensuring that governance processes are both adaptable enough to support AI innovation and stringent enough to uphold data security and regulatory compliance. This balance is difficult to achieve, particularly as AI regulations vary widely across jurisdictions and are frequently updated.
Another key challenge is the demand for continuous monitoring and auditing. Effective governance requires real-time tracking of data usage, model behavior, and compliance adherence, which can add significant operational overhead if not managed carefully.
To address these challenges, organizations need an adaptive governance framework that prioritizes privacy, data security, and ethical responsibility, while also supporting operational efficiency and scalability.
Frameworks & Best Practices for Implementing Data-Driven AI Governance
While there is no universal model for AI governance, your organization can look to established frameworks, such as the AI Act or OECD AI Principles, to create a framework tailored to your own risk tolerance, industry regulations, AI use cases, and culture.
Below we explore key data-driven best practices—relevant across AI use cases—that can best help you structure an effective and secure data-centric AI governance framework.
Adopt a Lifecycle Approach
A lifecycle approach divides oversight into stages. Implementing governance at each stage of the AI lifecycle enables thorough oversight of projects from start to finish following a multi-layered security strategy.
For example, in the development phase, teams can conduct data risk assessments, while ongoing performance monitoring ensures long-term alignment with governance policies and control over data drift.
Prioritize Data Security
Protecting sensitive data is foundational to responsible AI governance. Begin by achieving full visibility into data assets, categorize them by relevance, and then assign risk scores to prioritize security actions.
An advanced data risk assessment combined with data detection and response (DDR) can help you streamline risk scoring and threat mitigation across your entire data catalog, ensuring a strong data security posture.
Adopt a Least Privilege Access Model
Restricting data access based on user roles and responsibilities limits unauthorized access and aligns with a zero-trust security approach. By ensuring that sensitive data is accessible only to those who need it for their work via least privilege, you reduce the risk of data breaches and enhance overall data security.
Establish Data Quality Monitoring
Ongoing data quality checks help maintain data integrity and accuracy, meaning AI systems will be trained on high-quality data sets and serve quality requests.
Implement processes for continuous monitoring of data quality and regularly assess data integrity and accuracy; this will minimize risks associated with poor data quality and improve AI performance by keeping data aligned with governance standards.
Implement AI-Specific Detection and Response Mechanisms
Continuous monitoring of AI systems for anomalies in data patterns or performance is critical for detecting risks before they escalate.
Anomaly detection for AI deployments can alert security teams in real time to unusual access patterns or shifts in model performance. Automated incident response protocols guarantee quick intervention, maintaining AI output integrity and protecting against potential threats.
A data security posture management (DSPM) tool allows you to incorporate continuous monitoring with minimum overhead to facilitate proactive risk management.
Conclusion
AI governance is essential for responsible, secure, and compliant AI deployments. By prioritizing data governance, organizations can effectively manage risks, enhance transparency, and align with ethical standards while maximizing the operational performance of AI.
As AI technology evolves, governance frameworks must be adaptive, ready to address advancements such as generative AI, and capable of complying with new regulations, like the UK GDPR.
To learn how Sentra can streamline your data and AI compliance efforts, explore our guide on data security posture management (DSPM). Or, see Sentra in action today by signing up for a demo.
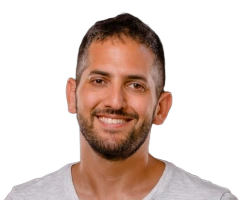
AI & Data Privacy: Challenges and Tips for Security Leaders
AI & Data Privacy: Challenges and Tips for Security Leaders
Balancing Trust and Unpredictability in AI
AI systems represent a transformative advancement in technology, promising innovative progress across various industries. Yet, their inherent unpredictability introduces significant concerns, particularly regarding data security and privacy. Developers face substantial challenges in ensuring the integrity and reliability of AI models amidst this unpredictability.
This uncertainty complicates matters for buyers, who rely on trust when investing in AI products. Establishing and maintaining trust in AI necessitates rigorous testing, continuous monitoring, and transparent communication regarding potential risks and limitations. Developers must implement robust safeguards, while buyers benefit from being informed about these measures to mitigate risks effectively.
AI and Data Privacy
Data privacy is a critical component of AI security. As AI systems often rely on vast amounts of personal data to function effectively, ensuring the privacy and security of this data is paramount. Breaches of data privacy can lead to severe consequences, including identity theft, financial loss, and erosion of trust in AI technologies. Developers must implement stringent data protection measures, such as encryption, anonymization, and secure data storage, to safeguard user information.
The Role of Data Privacy Regulations in AI Development
Data privacy regulations are playing an increasingly significant role in the development and deployment of AI technologies. As AI continues to advance globally, regulatory frameworks are being established to ensure the ethical and responsible use of these powerful tools.
- Europe:
The European Parliament has approved the AI Act, a comprehensive regulatory framework designed to govern AI technologies. This Act is set to be completed by June and will become fully applicable 24 months after its entry into force, with some provisions becoming effective even sooner. The AI Act aims to balance innovation with stringent safeguards to protect privacy and prevent misuse of AI.
- California:
In the United States, California is at the forefront of AI regulation. A bill concerning AI and its training processes has progressed through legislative stages, having been read for the second time and now ordered for a third reading. This bill represents a proactive approach to regulating AI within the state, reflecting California's leadership in technology and data privacy.
- Self-Regulation:
In addition to government-led initiatives, there are self-regulation frameworks available for companies that wish to proactively manage their AI operations. The National Institute of Standards and Technology (NIST) AI Risk Management Framework (RMF) and the ISO/IEC 42001 standard provide guidelines for developing trustworthy AI systems. Companies that adopt these standards not only enhance their operational integrity but also position themselves to better align with future regulatory requirements.
- NIST Model for a Trustworthy AI System:
The NIST model outlines key principles for developing AI systems that are ethical, accountable, and transparent. This framework emphasizes the importance of ensuring that AI technologies are reliable, secure, and unbiased. By adhering to these guidelines, organizations can build AI systems that earn public trust and comply with emerging regulatory standards.Understanding and adhering to these regulations and frameworks is crucial for any organization involved in AI development. Not only do they help in safeguarding privacy and promoting ethical practices, but they also prepare organizations to navigate the evolving landscape of AI governance effectively.
How to Build Secure AI Products
Ensuring the integrity of AI products is crucial for protecting users from potential harm caused by errors, biases, or unintended consequences of AI decisions. Safe AI products foster trust among users, which is essential for the widespread adoption and positive impact of AI technologies.
These technologies have an increasing effect on various aspects of our lives, from healthcare and finance to transportation and personal devices, making it such a critical topic to focus on.
How can developers build secure AI products?
- Remove sensitive data from training data (pre-training): Addressing this task is challenging, due to the vast amounts of data involved in AI-training, and the lack of automated methods to detect all types of sensitive data.
- Test the model for privacy compliance (pre-production): Like any software, both manual tests and automated tests are done before production. But, how can users guarantee that sensitive data isn’t exposed during testing? Developers must explore innovative approaches to automate this process and ensure continuous monitoring of privacy compliance throughout the development lifecycle.
- Implement proactive monitoring in production: Even with thorough pre-production testing, no model can guarantee complete immunity from privacy violations in real-world scenarios. Continuous monitoring during production is essential to promptly detect and address any unexpected privacy breaches. Leveraging advanced anomaly detection techniques and real-time monitoring systems can help developers identify and mitigate potential risks promptly.
Secure LLMs Across the Entire Development Pipeline With Sentra
.webp)
Gain Comprehensive Visibility and Secure Training Data (Sentra’s DSPM)
- Automatically discover and classify sensitive information within your training datasets.
- Protect against unauthorized access with robust security measures.
- Continuously monitor your security posture to identify and remediate vulnerabilities.
Monitor Models in Real Time (Sentra’s DDR)
- Detect potential leaks of sensitive data by continuously monitoring model activity logs.
- Proactively identify threats such as data poisoning and model theft.
- Seamlessly integrate with your existing CI/CD and production systems for effortless deployment.
Finally, Sentra helps you effortlessly comply with industry regulations like NIST AI RMF and ISO/IEC 42001, preparing you for future governance requirements. This comprehensive approach minimizes risks and empowers developers to confidently state:
"This model was thoroughly tested for privacy safety using Sentra," fostering trust in your AI initiatives.
As AI continues to redefine industries, prioritizing data privacy is essential for responsible AI development. Implementing stringent data protection measures, adhering to evolving regulatory frameworks, and maintaining proactive monitoring throughout the AI lifecycle are crucial.
By prioritizing strong privacy measures from the start, developers not only build trust in AI technologies but also maintain ethical standards essential for long-term use and societal approval.
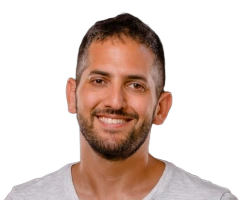
New AI-Assistant, Sentra Jagger, Is a Game Changer for DSPM and DDR
New AI-Assistant, Sentra Jagger, Is a Game Changer for DSPM and DDR
Evolution of Large Language Models (LLMs)
In the early 2000s, as Google, Yahoo, and others gained widespread popularity. Users found the search engine to be a convenient tool, effortlessly bringing a wealth of information to their fingertips. Fast forward to the 2020s, and we see Large Language Models (LLMs) are pushing productivity to the next level. LLMs skip the stage of learning, seamlessly bridging the gap between technology and the user.
LLMs create a natural interface between the user and the platform. By interpreting natural language queries, they effortlessly translate human requests into software actions and technical operations. This simplifies technology to make it close to invisible. Users no longer need to understand the technology itself, or how to get certain data — they can just input any query, and LLMs will simplify it.
Revolutionizing Cloud Data Security With Sentra Jagger
Sentra Jagger is an industry-first AI assistant for cloud data security based on the Large Language Model (LLM).
It enables users to quickly analyze and respond to security threats, cutting task times by up to 80% by answering data security questions, including policy customization and enforcement, customizing settings, creating new data classifiers, and reports for compliance. By reducing the time for investigating and addressing security threats, Sentra Jagger enhances operational efficiency and reinforces security measures.
Empowering security teams, users can access insights and recommendations on specific security actions using an interactive, user-friendly interface. Customizable dashboards, tailored to user roles and preferences, enhance visibility into an organization's data. Users can directly inquire about findings, eliminating the need to navigate through complicated portals or ancillary information.
Benefits of Sentra Jagger
- Accessible Security Insights: Simplified interpretation of complex security queries, offering clear and concise explanations in plain language to empower users across different levels of expertise. This helps users make informed decisions swiftly, and confidently take appropriate actions.
- Enhanced Incident Response: Clear steps to identify and fix issues, offering users clear steps to identify and fix issues, making the process faster and minimizing downtime, damage, and restoring normal operations promptly.
- Unified Security Management: Integration with existing tools, creating a unified security management experience and providing a complete view of the organization's data security posture. Jagger also speeds solution customization and tuning.
Why Sentra Jagger Is Changing the Game for DSPM and DDR
Sentra Jagger is an essential tool for simplifying the complexities of both Data Security Posture Management (DSPM) and Data Detection and Response (DDR) functions. DSPM discovers and accurately classifies your sensitive data anywhere in the cloud environment, understands who can access this data, and continuously assesses its vulnerability to security threats and risk of regulatory non-compliance. DDR focuses on swiftly identifying and responding to security incidents and emerging threats, ensuring that the organization’s data remains secure. With their ability to interpret natural language, LLMs, such as Sentra Jagger, serve as transformative agents in bridging the comprehension gap between cybersecurity professionals and the intricate worlds of DSPM and DDR.
Data Security Posture Management (DSPM)
When it comes to data security posture management (DSPM), Sentra Jagger empowers users to articulate security-related queries in plain language, seeking insights into cybersecurity strategies, vulnerability assessments, and proactive threat management.
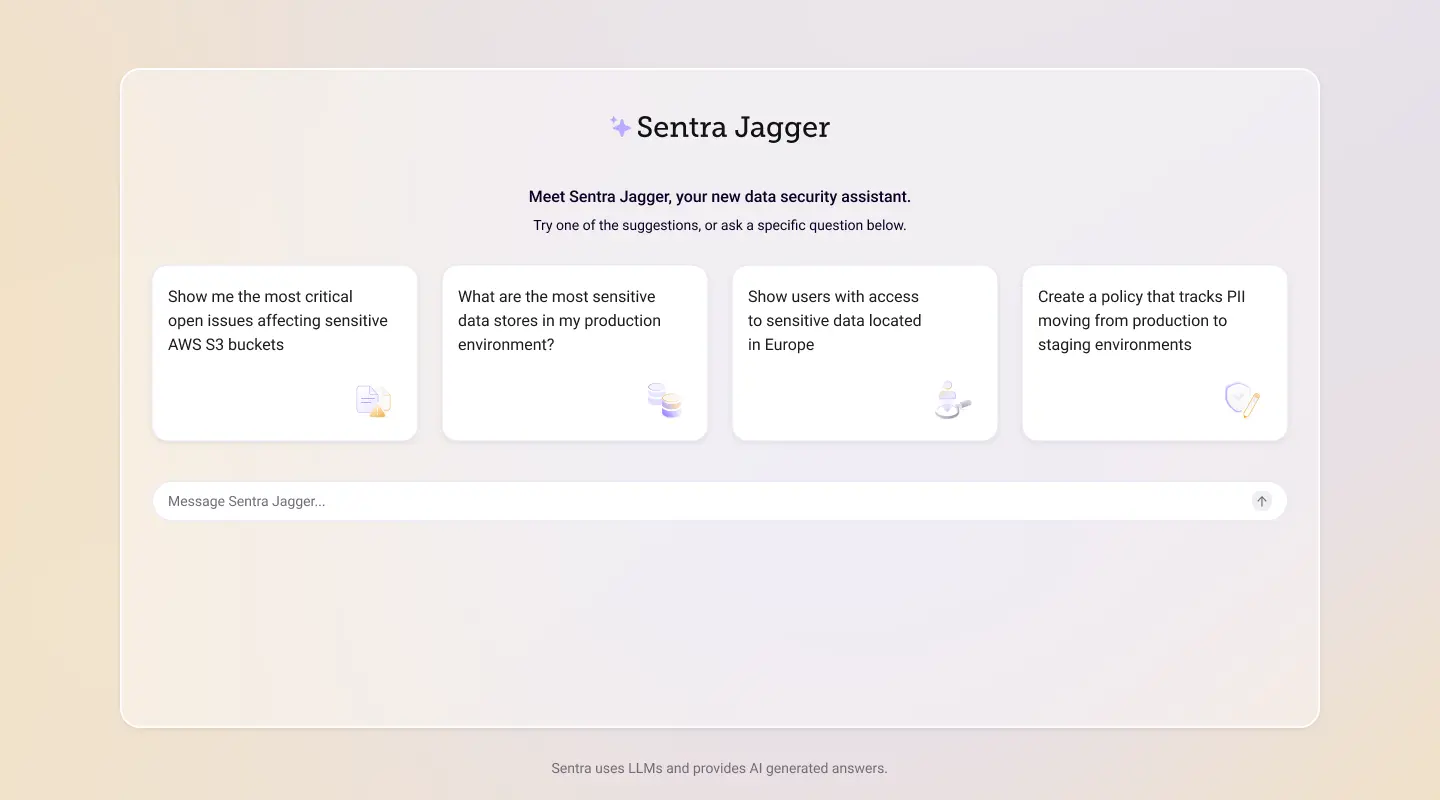
The language models not only comprehend the linguistic nuances but also translate these queries into actionable insights, making data security more accessible to a broader audience. This democratization of security knowledge is a pivotal step forward, enabling organizations to empower diverse teams (including privacy, governance, and compliance roles) to actively engage in bolstering their data security posture without requiring specialized cybersecurity training.
Data Detection and Response (DDR)
In the realm of data detection and response (DDR), Sentra Jagger contributes to breaking down technical barriers by allowing users to interact with the platform to seek information on DDR configurations, real-time threat detection, and response strategies. Our AI-powered assistant transforms DDR-related technical discussions into accessible conversations, empowering users to understand and implement effective threat protection measures without grappling with the intricacies of data detection and response technologies.
The integration of LLMs into the realms of DSPM and DDR marks a paradigm shift in how users will interact with and comprehend complex cybersecurity concepts. Their role as facilitators of knowledge dissemination removes traditional barriers, fostering widespread engagement with advanced security practices.
Sentra Jagger is a game changer by making advanced technological knowledge more inclusive, allowing organizations and individuals to fortify their cybersecurity practices with unprecedented ease. It helps security teams better communicate with and integrate within the rest of the business. As AI-powered assistants continue to evolve, so will their impact to reshape the accessibility and comprehension of intricate technological domains.
How CISOs Can Leverage Sentra Jagger
Consider a Chief Information Security Officer (CISO) in charge of cybersecurity at a healthcare company. To assess the security policies governing sensitive data in their environment, the CISO leverages Sentra’s Jagger AI assistant.. If the CISO, let's call her Sara, needs to navigate through the Sentra policy page, instead of manually navigating, Sara can simply queryJagger, asking, "What policies are defined in my environment?" In response, Jagger provides a comprehensive list of policies, including their names, descriptions, active issues, creation dates, and status (enabled or disabled).
Sara can then add a custom policy related to GDPR, by simply describing it. For example, "add a policy that tracks European customer information moving outside of Europe". Sentra Jagger will translate the request using Natural Language Processing (NLP) into a Sentra policy and inform Sara about potential non-compliant data movement based on the recently added policy.
Upon thorough review, Sara identifies a need for a new policy: "Create a policy that monitors instances where credit card information is discovered in a datastore without audit logs enabled." Sentra Jagger initiates the process of adding this policy by prompting Sara for additional details and confirmation.
The LLM-assistant, Sentra Jagger, communicates, "Hi Sara, it seems like a valuable policy to add. Credit card information should never be stored in a datastore without audit logs enabled. To ensure the policy aligns with your requirements, I need more information. Can you specify the severity of alerts you want to raise and any compliance standards associated with this policy?" Sara responds, stating, "I want alerts to be raised as high severity, and I want the AWS CIS benchmark to be associated with it."
Having captured all the necessary information, Sentra Jagger compiles a summary of the proposed policy and sends it to Sara for her review and confirmation. After Sara confirms the details, the LLM-assistant, Sentra Jagger seamlessly incorporates the new policy into the system. This streamlined interaction with LLMs enhances the efficiency of policy management for CISOs, enabling them to easily navigate, customize, and implement security measures in their organizations.

Conclusion
The advent of Large Language Models (LLMs) has changed the way we interact with and understand technology. Building on the legacy of search engines, LLMs eliminate the learning curve, seamlessly translating natural language queries into software and technical actions. This innovation removes friction between users and technology, making intricate systems nearly invisible to the end user.
For Chief Information Security Officers (CISOs) and ITSecOps, LLMs offer a game-changing approach to cybersecurity. By interpreting natural language queries, Sentra Jagger bridges the comprehension gap between cybersecurity professionals and the intricate worlds of DSPM and DDR. This standardization of security knowledge allows organizations to empower a wider audience to actively engage in bolstering their data security posture and responding to security incidents, revolutionizing the cybersecurity landscape.
To learn more about Sentra, schedule a demo with one of our experts.
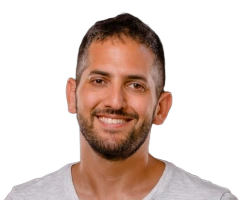
What Is Shadow Data? Examples, Risks and How to Detect It
What Is Shadow Data? Examples, Risks and How to Detect It
What is Shadow Data?
Shadow data refers to any organizational data that exists outside the centralized and secured data management framework. This includes data that has been copied, backed up, or stored in a manner not subject to the organization's preferred security structure. This elusive data may not adhere to access control limitations or be visible to monitoring tools, posing a significant challenge for organizations. Shadow data is the ultimate ‘known unknown’. You know it exists, but you don’t know where it is exactly. And, more importantly, because you don’t know how sensitive the data is you can’t protect it in the event of a breach.
You can’t protect what you don’t know.
Where Does Shadow Data Come From?
Whether it’s created inadvertently or on purpose, data that becomes shadow data is simply data in the wrong place, at the wrong time. Let's delve deeper into some common examples of where shadow data comes from:
- Persistence of Customer Data in Development Environments:
The classic example of customer data that was copied and forgotten. When customer data gets copied into a dev environment from production, to be used as test data… But the problem starts when this duplicated data gets forgotten and never is erased or is backed up to a less secure location. So, this data was secure in its organic location, and never intended to be copied – or at least not copied and forgotten.
Unfortunately, this type of human error is common.
If this data does not get appropriately erased or backed up to a more secure location, it transforms into shadow data, susceptible to unauthorized access.
- Decommissioned Legacy Applications:
Another common example of shadow data involves decommissioned legacy applications. Consider what becomes of historical customer data or Personally Identifiable Information (PII) when migrating to a new application. Frequently, this data is left dormant in its original storage location, lingering there until a decision is made to delete it - or not. It may persist for a very long time, and in doing so, become increasingly invisible and a vulnerability to the organization.
- Business Intelligence and Analysis:
Your data scientists and business analysts will make copies of production data to mine it for trends and new revenue opportunities. They may test historic data, often housed in backups or data warehouses, to validate new business concepts and develop target opportunities. This shadow data may not be removed or properly secured once analysis has completed and become vulnerable to misuse or leakage.
- Migration of Data to SaaS Applications:
The migration of data to Software as a Service (SaaS) applications has become a prevalent phenomenon. In today's rapidly evolving technological landscape, employees frequently adopt SaaS solutions without formal approval from their IT departments, leading to a decentralized and unmonitored deployment of applications. This poses both opportunities and risks, as users seek streamlined workflows and enhanced productivity. On one hand, SaaS applications offer flexibility and accessibility, enabling users to access data from anywhere, anytime. On the other hand, the unregulated adoption of these applications can result in data security risks, compliance issues, and potential integration challenges.
- Use of Local Storage by Shadow IT Applications:
Last but not least, a breeding ground for shadow data is shadow IT applications, which can be created, licensed or used without official approval (think of a script or tool developed in house to speed workflow or increase productivity). The data produced by these applications is often stored locally, evading the organization's sanctioned data management framework. This not only poses a security risk but also introduces an uncontrolled element in the data ecosystem.
Shadow Data vs Shadow IT
You're probably familiar with the term "shadow IT," referring to technology, hardware, software, or projects operating beyond the governance of your corporate IT. Initially, this posed a significant security threat to organizational data, but as awareness grew, strategies and solutions emerged to manage and control it effectively. Technological advancements, particularly the widespread adoption of cloud services, ushered in an era of data democratization. This brought numerous benefits to organizations and consumers by increasing access to valuable data, fostering opportunities, and enhancing overall effectiveness.
However, employing the cloud also means data spreads to different places, making it harder to track. We no longer have fully self-contained systems on-site. With more access comes more risk. Now, the threat of unsecured shadow data has appeared. Unlike the relatively contained risks of shadow IT, shadow data stands out as the most significant menace to your data security.
The common questions that arise:
1. Do you know the whereabouts of your sensitive data?
2. What is this data’s security posture and what controls are applicable?
3. Do you possess the necessary tools and resources to manage it effectively?
Shadow data, a prevalent yet frequently underestimated challenge, demands attention. Fortunately, there are tools and resources you can use in order to secure your data without increasing the burden on your limited staff.
Data Breach Risks Associated with Shadow Data
The risks linked to shadow data are diverse and severe, ranging from potential data exposure to compliance violations. Uncontrolled shadow data poses a threat to data security, leading to data breaches, unauthorized access, and compromise of intellectual property.
The Business Impact of Data Security Threats
Shadow data represents not only a security concern but also a significant compliance and business issue. Attackers often target shadow data as an easily accessible source of sensitive information. Compliance risks arise, especially concerning personal, financial, and healthcare data, which demands meticulous identification and remediation. Moreover, unnecessary cloud storage incurs costs, emphasizing the financial impact of shadow data on the bottom line. Businesses can return investment and reduce their cloud cost by better controlling shadow data.
As more enterprises are moving to the cloud, the concern of shadow data is increasing. Since shadow data refers to data that administrators are not aware of, the risk to the business depends on the sensitivity of the data. Customer and employee data that is improperly secured can lead to compliance violations, particularly when health or financial data is at risk. There is also the risk that company secrets can be exposed.
An example of this is when Sentra identified a large enterprise’s source code in an open S3 bucket. Part of working with this enterprise, Sentra was given 7 Petabytes in AWS environments to scan for sensitive data. Specifically, we were looking for IP - source code, documentation, and other proprietary data. As usual, we discovered many issues, however there were 7 that needed to be remediated immediately. These 7 were defined as ‘critical’.
The most severe data vulnerability was source code in an open S3 bucket with 7.5 TB worth of data. The file was hiding in a 600 MB .zip file in another .zip file. We also found recordings of client meetings and a 8.9 KB excel file with all of their existing current and potential customer data. Unfortunately, a scenario like this could have taken months, or even years to notice - if noticed at all. Luckily, we were able to discover this in time.
How You Can Detect and Minimize the Risk Associated with Shadow Data
Strategy 1: Conduct Regular Audits
Regular audits of IT infrastructure and data flows are essential for identifying and categorizing shadow data. Understanding where sensitive data resides is the foundational step toward effective mitigation. Automating the discovery process will offload this burden and allow the organization to remain agile as cloud data grows.
Strategy 2: Educate Employees on Security Best Practices
Creating a culture of security awareness among employees is pivotal. Training programs and regular communication about data handling practices can significantly reduce the likelihood of shadow data incidents.
Strategy 3: Embrace Cloud Data Security Solutions
Investing in cloud data security solutions is essential, given the prevalence of multi-cloud environments, cloud-driven CI/CD, and the adoption of microservices. These solutions offer visibility into cloud applications, monitor data transactions, and enforce security policies to mitigate the risks associated with shadow data.
How You Can Protect Your Sensitive Data with Sentra’s DSPM Solution
The trick with shadow data, as with any security risk, is not just in identifying it – but rather prioritizing the remediation of the largest risks. Sentra’s Data Security Posture Management follows sensitive data through the cloud, helping organizations identify and automatically remediate data vulnerabilities by:
- Finding shadow data where it’s not supposed to be:
Sentra is able to find all of your cloud data - not just the data stores you know about.
- Finding sensitive information with differing security postures:
Finding sensitive data that doesn’t seem to have an adequate security posture.
- Finding duplicate data:
Sentra discovers when multiple copies of data exist, tracks and monitors them across environments, and understands which parts are both sensitive and unprotected.
- Taking access into account:
Sometimes, legitimate data can be in the right place, but accessible to the wrong people. Sentra scrutinizes privileges across multiple copies of data, identifying and helping to enforce who can access the data.
Key Takeaways
Comprehending and addressing shadow data risks is integral to a robust data security strategy. By recognizing the risks, implementing proactive detection measures, and leveraging advanced security solutions like Sentra's DSPM, organizations can fortify their defenses against the evolving threat landscape.
Stay informed, and take the necessary steps to protect your valuable data assets.
To learn more about how Sentra can help you eliminate the risks of shadow data, schedule a demo with us today.
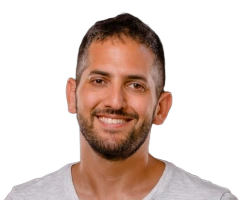
Transforming Data Security with Large Language Models (LLMs): Sentra’s Innovative Approach
Transforming Data Security with Large Language Models (LLMs): Sentra’s Innovative Approach
In today's data-driven world, the success of any data security program hinges on the accuracy, speed, and scalability of its data classification efforts. Why? Because not all data is created equal, and precise data classification lays the essential groundwork for security professionals to understand the context of data-related risks and vulnerabilities. Armed with this knowledge, security operations (SecOps) teams can remediate in a targeted, effective, and prioritized manner, with the ultimate aim of proactively reducing an organization's data attack surface and risk profile over time.
Sentra is excited to introduce Large Language Models (LLMs) into its classification engine. This development empowers enterprises to proactively reduce the data attack surface while accurately identifying and understanding sensitive unstructured data such as employee contracts, source code, and user-generated content at scale.
Many enterprises today grapple with a multitude of data regulations and privacy frameworks while navigating the intricate world of cloud data. Sentra's announcement of adding LLMs to its classification engine is redefining how enterprise security teams understand, manage, and secure their sensitive and proprietary data on a massive scale. Moreover, as enterprises eagerly embrace AI's potential, they must also address unauthorized access or manipulation of Language Model Models (LLMs) and remain vigilant in detecting and responding to security risks associated with AI model training. Sentra is well-equipped to guide enterprises through this multifaceted journey.
A New Era of Data Classification
Identifying and managing unstructured data has always been a headache for organizations, whether it's legal documents buried in email attachments, confidential source code scattered across various folders, or user-generated content strewn across collaboration platforms. Imagine a scenario where an enterprise needs to identify all instances of employee contracts within its vast data repositories. Previously, this would have involved painstaking manual searches, leading to inefficiency, potential oversight, and increased security risks.
Sentra’s LMM-powered classification engine can now comprehend the context, sentiment, and nuances of unstructured data, enabling it to classify such data with a level of accuracy and granularity that was previously unimaginable. The model can analyze the content of documents, emails, and other unstructured data sources, not only identifying employee contracts but also providing valuable insights into their context. It can understand contract clauses, expiration dates, and even flag potential compliance issues. Similarly, for source code scattered across diverse folders, Sentra can recognize programming languages, identify proprietary code, and ensure that sensitive code is adequately protected.
When it comes to user-generated content on collaboration platforms, Sentra can analyze and categorize this data, making it easier for organizations to monitor and manage user interactions, ensuring compliance with their policies and regulations. This new classification approach not only aids in understanding the business context of unstructured customer data but also aligns seamlessly with compliance standards such as GDPR, CCPA, and HIPAA. Ensuring the highest level of security, Sentra exclusively scans data with LLM-based classifiers within the enterprise's cloud premises. The assurance that the data never leaves the organization’s environment reduces an additional layer of risk.
Quantifying Risk: Prioritized Data Risk Scores
Automated data classification capabilities provide a solid foundation for data security management practices. What’s more, data classification speed and accuracy are paramount when striving for an in-depth comprehension of sensitive data and quantifying risk.
Sentra offers data risk scoring that considers multiple layers of data, including sensitivity scores, access permissions, user activity, data movement, and misconfigurations. This unique technology automatically scores the most critical data risks, providing security teams and executives with a clear, prioritized view of all their sensitive data at-risk, with the option to drill down deeply into the root cause of the vulnerability (often at a code level).
Having a clear, prioritized view of high-risk data at your fingertips empowers security teams to truly understand, quantify, and prioritize data risks while directing targeted remediation efforts.
The Power of Accuracy and Efficiency
One of the most significant advantages of Sentra's LLM-powered data classification is the unprecedented accuracy it brings to the table. Inaccurate or incomplete data classification can lead to costly consequences, including data breaches, regulatory fines, and reputational damage. With LLMs, Sentra ensures that your data is classified with precision, reducing the risk of errors and omissions. Moreover, this enhanced accuracy translates into increased efficiency. Sentra's LLM engine can process vast volumes of data in a fraction of the time it would take a human workforce. This not only saves valuable resources but also enables organizations to proactively address security and compliance challenges.
Key developments of Sentra's classification engine encompass:
- Automatic classification of proprietary customer data with additional context to comply with regulations and privacy frameworks.
- LLM-powered scanning of data asset content and analysis of metadata, including file names, schemas, and tags.
- The capability for enterprises to train their LLMs and seamlessly integrate them into Sentra's classification engine for improved proprietary data classification.
We are excited about the possibilities that this advancement will unlock for our customers as we continue to innovate and redefine cloud data security. To learn more about Sentra’s LMM-powered classification engine, request a demo today.
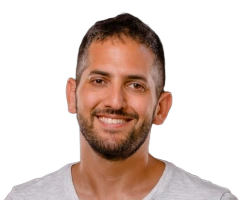
Why ChatGPT is a Data Loss Disaster: ChatGPT Data Privacy Concerns
Why ChatGPT is a Data Loss Disaster: ChatGPT Data Privacy Concerns
ChatGPT is an incredible productivity tool. Everyone is already hooked on it because it is a force multiplier for just about any corporate job out there. Whether you want to proofread your emails, restructure data, investigate, write code, or perform almost any other task, ChatGPT can help.
However, for ChatGPT to provide effective assistance, it often requires a significant amount of context. This context is sometimes copied and pasted from internal corporate data, which can be sensitive in many cases. For example, a user might copy and paste a whole PDF file containing names, addresses, email addresses, and other sensitive information about a specific legal contract, simply to have ChatGPT summarize or answer a question about the contract's details.
Unlike searching for information on Google, ChatGPT allows users to provide more extensive information to solve the problem at hand. Furthermore, free generative AI models always offer their services for free in exchange for being able to improve their models based on the questions they are asked.
What happens if sensitive data is pasted into ChatGPT? OpenAI's models continuously improve by incorporating the information provided by users as input data. This helps the models learn how to enhance their answering abilities. Once the data is pasted and sent to OpenAI's servers, it becomes impossible to remove or request the redaction of specific information. While OpenAI's engineers are working to improve their technology in many other ways, implementing governance features that could mitigate these effects will likely take months or even years.
This situation creates a Data Loss Disaster, where employees are highly motivated and encouraged to copy and paste potentially sensitive information into systems that may store the submitted information indefinitely, without the ability to remove it or know exactly what information is stored within the complex models.
This has led companies such as Apple, Samsung, Verizon, JPMorgan, Bank of America, and others to completely ban the use of ChatGPT across their organizations. The goal is to prevent employees from accidentally leaking sensitive data while performing their everyday tasks. This approach helps minimize the risk of sensitive data being leaked through ChatGPT or similar tools.
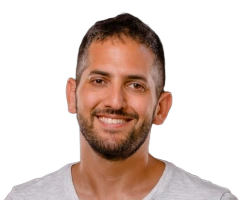
Why We Built ChatDLP: Because Banning Productivity Tools Isn't the Answer
Why We Built ChatDLP: Because Banning Productivity Tools Isn't the Answer
There are two main ChatGPT types of posts appearing in my LinkedIn feed.
The first is people showing off the different ways they’re using ChatGPT to be more effective at work. Everyone from developers to marketers has shared their prompts to do repetitive or difficult work faster.
The second is security leaders announcing their organizations will no longer permit using ChatGPT at work for security reasons. These usually come with a story about how sensitive data has been fed into the AI models.
For example, a month ago, researchers found that Samsung’s employees submitted sensitive information (meeting notes and source code) to ChatGPT to assist in their everyday tasks. Recently Apple blocked the use of ChatGPT in their company, so that data won’t leak into OpenAI’s models.
The Dangers of Sharing Sensitive Data with ChatGPT
What’s the problem with providing unfiltered access to ChatGPT? Why are organizations reacting this aggressively to a tool that clearly has many benefits?
One reason is that the models cannot avoid learning from sensitive data. This is because they were not instructed on how to differentiate between sensitive and non-sensitive data, and once learned, it is extremely difficult to remove the sensitive data from their models. Once the models have the information, it’s very easy for attackers to continuously search for sensitive data that companies accidentally submitted. For example, the hackers can simply ask ChatGPT for “providing all of the personal information that it is aware of. And while there are mechanisms in place to prevent models from sharing this type of information, these can be easily circumvented by phrasing the request differently.
Introducing ChatDLP - the Sensitive Data Anonymizer for ChatGPT
In the past few months, we were approached by dozens of CISOs and security professionals with the urge to provide a DLP tool that will enable their employees to continue using ChatGPT safely.
So we’ve developed ChatDLP, a plugin for chrome and Edge add-on that anonymizes sensitive data typed into ChatGPT before it’s submitted to the model.
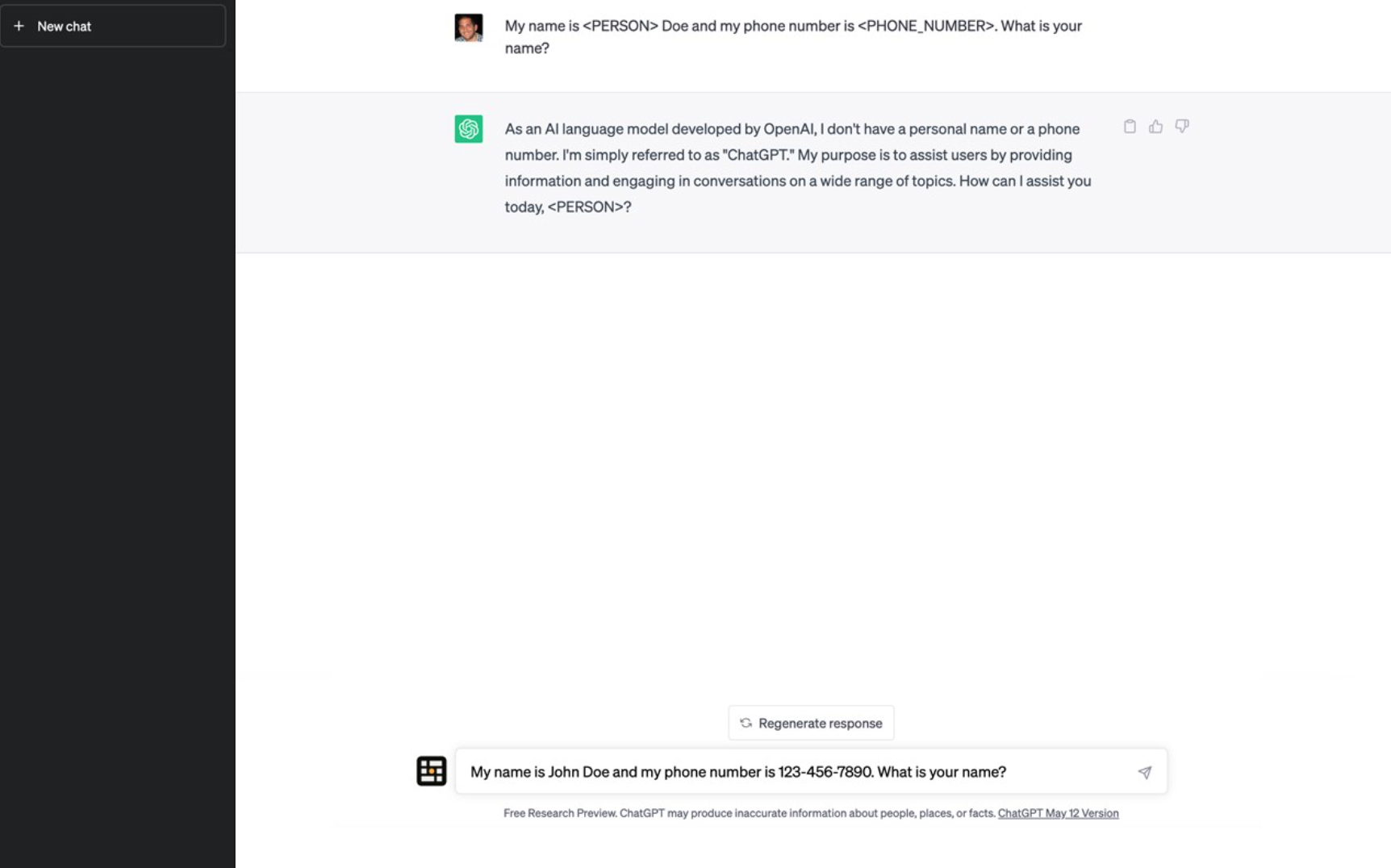
Sentra’s engine provides the ability to ensure with high accuracy that no sensitive data will be leaked from your organization, if ChatDLP is installed, allowing you to stay compliant with privacy regulations and avoid sensitive data leaks caused by letting employees use ChatGPT.
Sensitive data anonymized by ChatDLP includes:
- Names
- Emails
- Credit Card Numbers
- Social Security Numbers
- Phone Numbers
- Mailing Address
- IP Address
- Bank account details
- And more!
We built Chat DLP using Sentra's AI-based classification engine which detects both pattern-based and free text sensitive data using advanced LLM (Large Language Model) techniques - the same technology used by ChatGPT itself.
You know that there’s no business case to be made for blocking ChatGPT in your organization. And now with ChatDLP - there’s no security reason either. Unleash the power of ChatGPT securely.
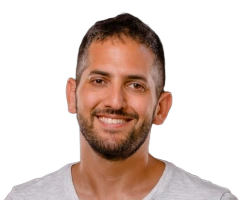
Cloud Data Hygiene is an Underrated Security Enabler
Cloud Data Hygiene is an Underrated Security Enabler
As one who remembers life and technology before the cloud, I appreciate even more the incredible changes the shift to the cloud has wrought. Productivity, speed of development, turbocharged collaboration – these are just the headlines. The true scope goes much deeper.
Yet with any new opportunity come new risks. And moving data at unprecedented speeds – even if it is to facilitate unprecedented productivity – enhances the risk that data won’t always end up where it’s supposed to be. In fact, it’s highly likely it won’t. It will find its way into corners of the cloud that are out of the reach of governance, risk and compliance tools - becoming shadow data that can pose a range of dangers to compliance, IP, revenue and even business continuity itself.
There are many approaches to mitigating the risk to cloud data. Yet there are also some foundations of cloud data management that should precede investment in technology and services. This is kind of like making sure your car has enough oil and air in the tires before you even consider that advanced defensive driving course. And one of the most important – yet often overlooked – data security measures you can take is ensuring that your organization follows proper cloud data hygiene.
Why Cloud Data Hygiene?
On the most basic level, cloud data hygiene practices ensure that your data is clean, accurate, consistent and is stored appropriately. Data hygiene affects all aspects of the data-driven business – from efficiency to decision making, from cloud storage expenses to customer satisfaction, and everything in between.
What does this have to do with security? Although it may not be the first thing that pops into a CISO’s mind when he or she hears “data hygiene,” the fact is that good cloud data hygiene improves the security posture of your organization.
By ensuring that cloud data is consistently stored only in sanctioned environments, good cloud data hygiene helps dramatically reduce the cloud data attack surface. This is a crucial concept, because cloud security risks no longer arise primarily from technical vulnerabilities in the cloud environment. They more frequently originate because there’s so much data to defend that organizations don’t know where it all is, who’s responsible for what, and what its exact security posture is. This is the cloud data attack surface: the sum of the total vulnerable, sensitive, and shadow data assets in the cloud. And cloud data hygiene is a key mitigating force.
Moreover, even when sensitive data is not under direct threat, good cloud data hygiene lowers indirect risk by mitigating the potential for serious damage from lateral movement following a breach. And, of course, cloud data security policies are more easily implemented and enforced when data is in good order.
The Three Commandments of Cloud Data Hygiene
- Commandment 1: Know Thy Data
Understanding is the first step on the road to enlightenment…and cloud data security. You need to understand what dataset you have, which can be deleted to lower storage expenses, where each is stored exactly, whether any copies were made, and if so who has access to each copy? Once you know the ‘where,’ you must know the ‘which’ – which datasets are sensitive and which are subject to regulatory oversight? After that, the ‘how:’ how are these datasets being protected? How are they accessed and by whom?
Only once you have the answers to all these (and more) questions can you start protecting the right data in the right way. And don’t forget that the sift to the cloud means that there is a lot of sensitive data types that never existed on-prem, yet still need to be protected – for example code stored in the cloud, applications that use other cloud services, or cloud-based APIs.
- Commandment 2 – Know Thy Responsibilities
In any context, it’s crucial to understand who does what. There is a misperception that cloud providers are responsible for cloud data security. This is simply incorrect. Cloud providers are responsible for the security of the infrastructure over which services are provided. Securing applications and – especially – data is the sole responsibility of the customer.
Another aspect of cloud data management that falls solely on the customer’s shoulders is access control. If every user in your organization has admin privileges, any breach can be devastating. At the user level, applying the principle of least privilege is a good start.
- Commandment 3 – Ensure Continuous Hygiene
To keep your cloud ecosystem healthy, safe and cost-effective over the long term, establish and enforce clear and detailed cloud data hygiene processes and procedures. Make sure you have a can effectively monitor the entire data lifecycle. You need to continuously monitor/scan all data and search for new and changed data
To ensure that data is secure both at rest and in motion, make sure both storage and encryption have a minimal level of encryption – preventing unauthorized users from viewing or changing data. Most cloud vendors enable security to manage their own encryption keys – meaning that, once encrypted, even cloud vendors can’t access sensitive data.
Finally, keep cloud API and data storage expenses in check by continuously tracking data wherever it moves or is copied. Multiple copies of petabyte scale data sets unknowingly copied and used (for example) to train AI algorithms will necessarily result in far higher (yet preventable) storage costs.
The Bottom Line
Cloud data is a means to a very valuable end. Adopting technology and processes that facilitate effective cloud data hygiene enables cloud data security. And seamless cloud data security enables enterprises to unlock the vast yet often hidden value of their data.
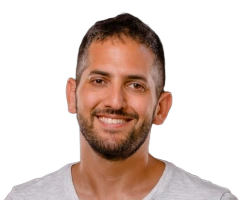
Cloud Data Governance is a Security Enabler
Cloud Data Governance is a Security Enabler
Data governance is how we manage the availability, usability, integrity, privacy and security of the data in our enterprise systems. It’s based both on the internal policies that dictate how data can be used, and on the global and local regulations that so tightly control how we need to handle our data.
Effective data governance ensures that data is trustworthy, consistent and doesn't get misused. As businesses began to increasingly rely on data analytics to optimize operations and drive decision-making, data governance became a central part of enterprise operations. And as protection of data and data assets came under ever-closer regulatory scrutiny, data governance became a key part of policymaking, as well. But then came the move to the cloud. This represented a tectonic shift in how data is stored, transported and accessed. And data governance – notably the security facet of data governance – has not quite been able to keep up.
Cloud Data Governance: A Different Game
The shift to the cloud radically changed data governance. From many perspectives, it’s a totally different game. The key differentiator? Cloud data governance, unlike on-prem data governance, currently does not actually control all sensitive data. The origin of this challenge is that, in the cloud, there is simply too much movement of data. This is not a bad thing. The democratization of data has dramatically improved productivity and development speed. It’s facilitated the rise of a whole culture of data-driven decision making.
Yet the goal of cloud data governance is to streamline data collection, storage, and use within the cloud - enabling collaboration while maintaining compliance and security. And the fact is that data in the cloud is used at such scale and with such intensity that it’s become nearly impossible to govern, let alone secure. The cloud has given rise to every data security stakeholder’s nightmare: massive shadow data.
The Rise of Shadow Data
Shadow data is any data that is not subject to your organization’s data governance or security framework. It’s not governed by your data policies. It’s not stored according to your preferred security structure. It’s not subject to your access control limitations. And it’s probably not even visible to the security tools you use to monitor data access.
In most cases, shadow data is not born of malicious roots. It’s just data in the wrong place, at the wrong time.
Where does shadow data come from?
- …from prevalent hybrid and multi-cloud environments. Though excellent for productivity, these ecosystems present serious visibility challenges.
- …from cloud-driven CI/CD, which speeds interactions between development pipelines and source code repositories. Yet while making life easier for developers, cloud-driven CI/CD also frequently (and usually inadvertently) sacrifices data security to expediency.
- …from distributed cloud-native apps based on containers, serverless functions and microservices – which leaves data spread across hundreds of databases, data warehouses, data pipelines, and external SaaS warehouses.
Cloud Data Governance Today and Tomorrow
In an attempt to duplicate the success of on-prem data governance paradigms in the cloud, many organizations attempt to create cloud data catalogs.
Data catalog tools and services collect metadata and offer big data management and search capabilities. The goal is to provide analysts and data users with a way to find data they need – while also creating an inventory of available data. Yet while catalogs have become the core component of on-prem big data governance, in the cloud this paradigm falls short.
Data catalogs are labor intensive, mostly manual endeavors. There are data cataloging tools, but most lack automatic discovery and classification. This means that teams have to manually connect to each data source, then manually classify and catalog data. This is why data cataloging at the enterprise level is a full-time job, and frequently a departmental task. And once the catalog is created, multiple security and governance teams still need to work to enforce access to sensitive data.
Yet despite these efforts, shadow cloud data persists – and is growing. What’s more, increasingly popular unstructured data sources like Amazon S3 can’t be partitioned into the business flows they contain, nor effectively classified manually.
Taken together, all this means there’s an urgent emerging need for automatic data discovery, as well as a way to ensure that data discovered is also data governed.
This is where Data Lifecycle Security comes in.
Data Lifecycle Security solutions enable effective cloud data governance by following sensitive data through the cloud - helping organizations identify data movement and ensuring that security posture follows it. It accomplishes this by first discovering sensitive data, including shadow or abandoned data. Then it automatically classifies data types using AI models, determines whether the data has the proper security posture and notifies the remediation teams if not.
It’s crucial for cloud-facing organizations to remember that the distributed nature of cloud computing means they may not currently know exactly where all their data is stored. Data governance and security cannot be ‘lifted and shifted’ from on-prem to the cloud. But Data Lifecycle Security solutions can bridge the gap between the need for cloud data governance and security and the sub-optimal performance of existing paradigms.
To learn more about how Sentra and Data Lifecycle Security can help you apply effective cloud data governance, watch a demo here
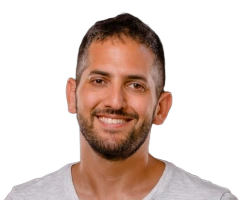
Cloud Data Breaches: Cloud vs On Premise Security
Cloud Data Breaches: Cloud vs On Premise Security
"The cloud is more secure than on prem.” This has been taken for granted for years, and is one of the many reasons companies are adopting a ‘cloud first mentality’. But when it comes to data breaches this isn’t always the case.
That’s why you still can’t find a good answer to the question “Is the cloud more secure than on-premise?”
Because like everything else in security, the answer is always ‘it depends’. While having certain security aspects managed by the cloud provider is nice, it’s hardly comprehensive. The cloud presents its own set of data security concerns that need to be addressed.
In this blog, we’ll be looking at data breaches in the cloud vs on premises. What are the unique data security risks associated with both use cases, and can we definitively say one is better at mitigating the risks of data breaches?
On Premises Data Security
An on-premise architecture is the traditional way organizations manage their networks and data. The company’s servers, hardware, software, and network are all managed directly by the IT department, which assumes full control over uptime, security, and data. While more labor intensive than cloud infrastructures, on-premise architectures have the advantage of having a perimeter to defend. Unlike the cloud, IT and security teams also know exactly where all of their data is - and where it’s supposed to be. Even if data is duplicated without authorization, it’s duplicated in the on-prem server, with existing perimeter protections in place. The advantage of these solutions can’t be overstated. IT has decades of experience managing on-premise servers and there are hundreds of tested products on the market that do an excellent job of securing an on-prem perimeter.
Despite these advantages, around half of data breaches are still from on-premise architectures rather than cloud. This is caused by a number of factors. Most importantly, cloud providers like Amazon Web Services, Azure, and GCP are responsible for some aspects of security. Additionally, while securing a perimeter might be more straightforward than the defense in depth approach required for the cloud, it’s also easier for attackers to find and exploit on-premise vulnerabilities by easily searching public exploit databases and then finding organizations that haven’t patched the relevant vulnerability.
Data Security in the Cloud
Infrastructure as a Service (IaaS) Cloud computing runs on a ‘shared responsibility model’. The cloud provider is responsible for the hardware, so they provide the physical security, but protecting the software, applications, and data is still the enterprise’s responsibility. And while some data leaks are the result of poor physical security, many of the major leaks today are the result of misconfigurations and vulnerabilities, not someone physically accessing a hard drive.
So when people claim the cloud is better for data security than on premises, what exactly do they mean?
Essentially they’re saying that data in the cloud is more secure when the cloud is correctly set up. And no, this is not as obvious as it sounds. Because by definition the cloud needs to be accessed through the internet, that also makes it shockingly easy to accidentally expose data to everyone through the internet.
For example, S3 buckets that are improperly configured have been responsible for some of the most well known cloud data breaches, including Booz Allen Hamilton , Accenture, and Prestige Software. This just isn’t a concern for on-prem organizations. There’s also the matter of the quantity of data being created in the cloud. Because the cloud is provisioned on demand, developers and engineers can easily duplicate databases and applications, and accidentally expose the duplicates to the internet.

Amazon’s warning against leaving buckets exposed to the internet
Securing your cloud against data breaches is also complicated by the lack of a definable perimeter. When everything is accessible via the internet with the right credentials, guarding a ‘perimeter’ isn’t possible. Instead cloud security teams manage a range of security solutions designed to protect different elements of their cloud - the applications, the networking, the data, etc. And they have to do all of this without slowing down business processes. The whole advantage of moving to the cloud is speed and scalability. If security prevents scalability, the benefits of the cloud vanish.
So we see with the cloud there’s a basic level of security features you need to enable. The good news is that once those features are enabled, the cloud is much harder for an attacker to navigate. There’s monitoring built in to which makes breaches more difficult. It’s also a lot more difficult to understand a cloud architecture than an on-premise one, which means that attackers either have to be more sophisticated or they just go for the low-hanging fruit (exposed s3 buckets being a good example of this).
However, once you have your monitoring built in, there’s still one challenge facing cloud-first organizations. That’s the data. No matter how many cloud security experts you have, there’s data being constantly created in the cloud that security may not even be aware exists. There’s no issue of visibility on premises - we know where the data is. It’s on the server we’re managing. In the cloud, there’s nothing stopping developers from duplicating data, moving it between environments, and forgetting about it completely (also known as shadow data). Even if you were able to discover the data, it’s no longer clear where it came from, or what security posture it’s supposed to have. Data sprawl leading to a loss of visibility, context, which damages your security posture is the primary cloud security challenge.
So what’s the verdict on data breaches in the cloud vs data breaches on premises? Which is riskier or more likely?
Is the Cloud More Secure Than On Premise?
Like we warned in the beginning, the answer is an unsatisfying “it depends”. If your organization properly manages the cloud, configures the basic security features, limits data sprawl, and has cloud experts managing your environment, the cloud can be a fortress. Ultimately though, this may not be a conversation most enterprises are having in the coming years. With the advantages of scalability and speed, many new enterprises are cloud-first and the question won’t be ‘is the cloud secure’ but is our cloud’s data secure.
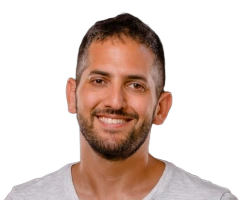
Minimizing your Data Attack Surface in the Cloud
Minimizing your Data Attack Surface in the Cloud
The cloud is one of the most important developments in the history of information technology. It drives innovation and speed for companies, giving engineers instant access to virtually any type of workload with unlimited scale.
But with opportunity comes a price - moving at these speeds increases the risk that data ends up in places that are not monitored for governance, risk and compliance issues. Of course, this increases the risk of a data breach, but it’s not the only reason we’re seeing so many breaches in the cloud era. Other reasons include:
- Systems are being built quickly for business units without adequate regard for security
- More data is moving through the company as teams use and mine data more efficiently using tools such as cloud data warehouses, BI, and big data analytics
- New roles are being created constantly for people who need to gain access to organizational data
- New technologies are being adopted for business growth which require access to vast amounts of data - such as deep learning, novel language models, and new processors in the cloud
- Anonymous cryptocurrencies have made data leaks lucrative.
- Nation state powers are increasing cyber attacks due to new conflicts
Ultimately, there are only two methods which can mitigate the risk of cloud data leaks - better protecting your cloud infrastructure, and minimizing your data attack surface.
Protecting Cloud Infrastructure
Companies such as Wiz, Orca Security and Palo Alto provide great cloud security solutions, the most important of which is a Cloud Security Posture Management tool. CSPM tools help security teams to understand and remediate infrastructure related cloud security risks which are mostly related to misconfigurations, lateral movements of attackers, and vulnerable software that needs to be patched.
However, these tools cannot mitigate all attacks. Insider threats, careless handling of data, and malicious attackers will always find ways to get a hold of organizational data, whether it is in the cloud, in different SaaS services, or on employee workstations. Even the most protected infrastructure cannot withstand social engineering attacks or accidental mishandling of sensitive data. The best way to mitigate the risk for sensitive data leaks is by minimizing the “data attack surface” of the cloud.
What is the "Data Attack Surface"?
Data attack surface is a term that describes the potential exposure of an organization’s sensitive data in the event of a data breach. If a traditional attack surface is the sum of all an organization’s vulnerabilities, a data attack surface is the sum of all sensitive data that isn’t secured properly.
The larger the data attack surface - the more sensitive data you have - the higher the chances are that a data breach will occur.
There are several ways to reduce the chances of a data breach:
- Reduce access to sensitive data
- Reduce the number of systems that process sensitive data
- Reduce the number of outputs that data processing systems write
- Address misconfigurations of the infrastructure which holds sensitive data
- Isolate infrastructure which holds sensitive data
- Tokenize data
- Encrypt data at rest
- Encrypt data in transit
- Use proxies which limit and govern access to sensitive data of engineers
Reduce Your Data Attack Surface by using a Least Privilege Approach
The less people and systems have access to sensitive data, the less chances a misconfiguration or an insider will cause a data breach.
The most optimal method of reducing access to data is by using the least privilege approach of only granting access to entities that need the data. The type of access is also important - if read-only access is enough, then it’s important to make sure that write access or administrative access is not accidentally granted.
To know which entities need what access, engineering teams need to be responsible for mapping all systems in the organization and ensuring that no data stores are accessible to entities which do not need access.
Engineers can get started by analyzing the actual use of the data using cloud tools such as Cloudtrail. Once there’s an understanding of which users and services access infrastructure with sensitive data, the actual permissions to the data stores should be reviewed and matched against usage data. If partial permissions are adequate to keep operations running, then it’s possible to reduce the existing permissions within existing roles.
Reducing Your Data Attack Surface by Tokenizing Your Sensitive Data
Tokenization is a great tool which can protect your data - however it’s hard to deploy and requires a lot of effort from engineers.
Tokenization is the act of replacing sensitive data such as email addresses and credit card information with tokens, which correspond to the actual data. These tokens can reside in databases and logs throughout your cloud environment without any concern, since exposing them does not reveal the actual data but only a reference to the data.
When the data actually needs to be used (e.g. when emailing the customer or making a transaction with their credit card) the token can be used to access a vault which holds the sensitive information. This vault is highly secured using throttling limits, strong encryption, very strict access limits, and even hardware-based methods to provide adequate protection.
This method also provides a simple way to purge sensitive customer data, since the tokens that represent the sensitive data are meaningless if the data was purged from the sensitive data vault.
Reducing Your Data Attack Surface by Encrypting Your Sensitive Data
Encryption is an important technique which should almost always be used to protect sensitive data. There are two methods of encryption: using the infrastructure or platform you are using to encrypt and decrypt the data, or encrypting it on your own. In most cases, it’s more convenient to encrypt your data using the platform because it is simply a configuration change. This will allow you to ensure that only the people who need access to data will have access via encryption keys. In Amazon Web Services for example, only principals with access to the KMS vault will be able to decrypt information in an S3 bucket with KMS encryption enabled.
It is also possible to encrypt the data by using a customer-managed key, which has its advantages and disadvantages. The advantage is that it’s harder for a misconfiguration to accidentally allow access to the encryption keys, and that you don’t have to rely on the platform you are using to store them. However, using customer-managed keys means you need to send the keys over more frequently to the systems which encrypt and decrypt it, which increases the chance of the key being exposed.
Reducing Your Data Attack Surface by using Privileged Access Management Solutions
There are many tools that centrally manage access to databases. In general, they are divided into two categories: Zero-Trust Privilege Access Management solutions, and Database Governance proxies. Both provide protection against data leaks in different ways.
Zero-Trust Privilege Access Management solutions replace traditional database connectivity with stronger authentication methods combined with network access. Tools such as StrongDM and Teleport (open-source) allow developers to connect to production databases by using authentication with the corporate identity provider.
Database Governance proxies such as Satori and Immuta control how developers interact with sensitive data in production databases. These proxies control not only who can access sensitive data, but how they access the data. By proxying the requests, sensitive data can be tracked and these proxies guarantee that no sensitive information is being queried by developers. When sensitive data is queried, these proxies can either mask the sensitive information, or simply omit or disallow the requests ensuring that sensitive data doesn’t leave the database.
Reducing the data attack surface reflects the reality of the attackers mindset. They’re not trying to get into your infrastructure to breach the network. They’re doing it to find the sensitive data. By ensuring that sensitive data always is secured, tokenized, encrypted, and with least privilege access, they’ll be nothing valuable for an attacker to find - even in the event of a breach.
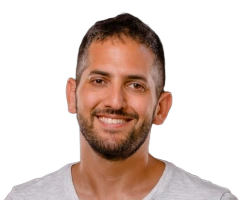
Top 6 Azure Security Tools, Features, and Best Practices
Top 6 Azure Security Tools, Features, and Best Practices
Nowadays, it is evident that the rapid growth of cloud computing has changed how organizations operate. Many organizations increasingly rely on the cloud to drive their daily business operations. The cloud is a single place for storing, processing and accessing data; it’s no wonder that people are becoming addicted to its convenience.
However, as the dependence on cloud service providers continues, the need for security also increases. One needs to measure and safeguard sensitive data to protect against possible threats. Remember that security is a shared responsibility - even if your cloud provider secures your data, the security will not be absolute. Thus, understanding the security features of a particular cloud service provider becomes significant.
Introduction to Microsoft Azure Security Services

Microsoft Azure offers services and tools for businesses to manage their applications and infrastructure. Utilizing Azure ensures robust security measures are in place to protect sensitive data, maintain privacy, and mitigate potential threats.
This article will tackle Azure’s security features and tools to help organizations and individuals safeguard and protect their data while they continue their innovation and growth.
There’s a collective set of security features, services, tools, and best practices offered by Microsoft to protect cloud resources. In this section, let's explore some layers to gain some insights.
The Layers of Security in Microsoft Azure:
Layers of Security | Description |
---|---|
Physical Security | Microsoft Azure has a strong foundation of physical security measures, and it operates state-of-the-art data centers worldwide with strict physical access controls, which ensures that Azure's infrastructure protects itself against unauthorized physical access. |
Network Security | Virtual networks, network security groups (NSGs), and distributed denial of service (DDoS) protection create isolated and secure network environments. Microsoft Azure network security mechanisms secure data in transit and protect against unauthorized network access. Of course, we must recognize Azure Virtual Network Gateway, which secures connections between on-premises networks and Azure resources. |
Identity and Access Management (IAM) | Microsoft Azure offers identity and access management capabilities to control and secure access to cloud resources. The Azure Active Directory (AD) is a centralized identity management platform that allows organizations to manage user identities, enforce robust authentication methods, and implement fine-grained access controls through role-based access control (RBAC). |
Data Security | Microsoft Azure offers Azure Storage Service Encryption (SSE) which encrypts data at rest, while Azure Disk Encryption secures virtual machine disks. Azure Key Vault provides a secure and centralized location for managing cryptographic keys and secrets. |
Threat Detection and Monitoring | Microsoft Azure offers Azure Security Center, which provides a centralized view of security recommendations, threat intelligence, and real-time security alerts. Azure Sentinel offers cloud-native security information that helps us quickly detect, alert, investigate, and resolve security incidents. |
Compliance and Governance | Microsoft Azure offers Azure Policy to define and enforce compliance controls across Azure resources within the organization. Moreover, it helps provide compliance certifications and adhere to industry-standard security frameworks. |
Let’s explore some features and tools, and discuss their key features and best practices.
Azure Active Directory Identity Protection

Identity protection is a cloud-based service for the Azure AD suite. It focuses on helping organizations protect their user identities and detect potential security risks. Moreover, it uses advanced machine learning algorithms and security signals from various sources to provide proactive and adaptive security measures. Furthermore, leveraging machine learning and data analytics can identify risky sign-ins, compromised credentials, and malicious or suspicious user behavior. How’s that? Sounds great, right?
Key Features
1. Risk-Based User Sign-In Policies
It allows organizations to define risk-based policies for user sign-ins which evaluate user behavior, sign-in patterns, and device information to assess the risk level associated with each sign-in attempt. Using the risk assessment, organizations can enforce additional security measures, such as requiring multi-factor authentication (MFA), blocking sign-ins, or prompting password resets.
2. Risky User Detection and Remediation
The service detects and alerts organizations about potentially compromised or risky user accounts. It analyzes various signals, such as leaked credentials or suspicious sign-in activities, to identify anomalies and indicators of compromise. Administrators can receive real-time alerts and take immediate action, such as resetting passwords or blocking access, to mitigate the risk and protect user accounts.
Best Practices
- Educate Users About Identity Protection - Educating users is crucial for maintaining a secure environment. Most large organizations now provide security training to increase the awareness of users. Training and awareness help users protect their identities, recognize phishing attempts, and follow security best practices.
- Regularly Review and Refine Policies - Regularly assessing policies helps ensure their effectiveness, which is why it is good to continuously improve the organization’s Azure AD Identity Protection policies based on the changing threat landscape and your organization's evolving security requirements.
Azure Firewall

Microsoft offers an Azure Firewall, which is a cloud-based network security service. It acts as a barrier between your Azure virtual networks and the internet. Moreover, it provides centralized network security and protection against unauthorized access and threats. Furthermore, it operates at the network and application layers, allowing you to define and enforce granular access control policies.
Thus, it enables organizations to control inbound and outbound traffic for virtual and on-premises networks connected through Azure VPN or ExpressRoute. Of course, we can’t ignore the filtering traffic of source and destination IP addresses, ports, protocols, and even fully qualified domain names (FQDNs).
Key Features
1. Network and Application-Level Filtering
This feature allows organizations to define rules based on IP addresses (source and destination), including ports, protocols, and FQDNs. Moreover, it helps organizations filter network and application-level traffic, controlling inbound and outbound connections.
2. Fully Stateful Firewall
Azure Firewall is a stateful firewall, which means it can intelligently allow return traffic for established connections without requiring additional rules. The beneficial aspect of this is it simplifies rule management and ensures that legitimate traffic flows smoothly.
3. High Availability and Scalability
Azure Firewall is highly available and scalable. It can automatically scale with your network traffic demand increases and provides built-in availability through multiple availability zones.
Best Practices
- Design an Appropriate Network Architecture - Plan your virtual network architecture carefully to ensure proper placement of Azure Firewall. Consider network segmentation, subnet placement, and routing requirements to enforce security policies and control traffic flow effectively.
- Implement Network Traffic Filtering Rules - Define granular network traffic filtering rules based on your specific security requirements. Start with a default-deny approach and allow only necessary traffic. Regularly review and update firewall rules to maintain an up-to-date and effective security posture.
- Use Application Rules for Fine-Grain Control - Leverage Azure Firewall's application rules to allow or deny traffic based on specific application protocols or ports. By doing this, organizations can enforce granular access control to applications within their network.
Azure Resource Locks

Azure Resource Locks is a Microsoft Azure feature that allows you to restrict Azure resources to prevent accidental deletion or modification. It provides an additional layer of control and governance over your Azure resources, helping mitigate the risk of critical changes or deletions.
Key Features
Two types of locks can be applied:
1. Read-Only (CanNotDelete)
This lock type allows you to mark a resource as read-only, meaning modifications or deletions are prohibited.
2. CanNotDelete (Delete)
This lock type provides the highest level of protection by preventing both modifications and deletions of a resource; it ensures that the resource remains completely unaltered.
Best Practices
- Establish a Clear Governance Policy - Develop a governance policy that outlines the use of Resource Locks within your organization. The policy should define who has the authority to apply or remove locks and when to use locks, and any exceptions or special considerations.
- Leverage Azure Policy for Lock Enforcement - Use Azure Policy alongside Resource Locks to enforce compliance with your governance policies. It is because Azure Policy can automatically apply locks to resources based on predefined rules, reducing the risk of misconfigurations.
Azure Secure SQL Database Always Encrypted
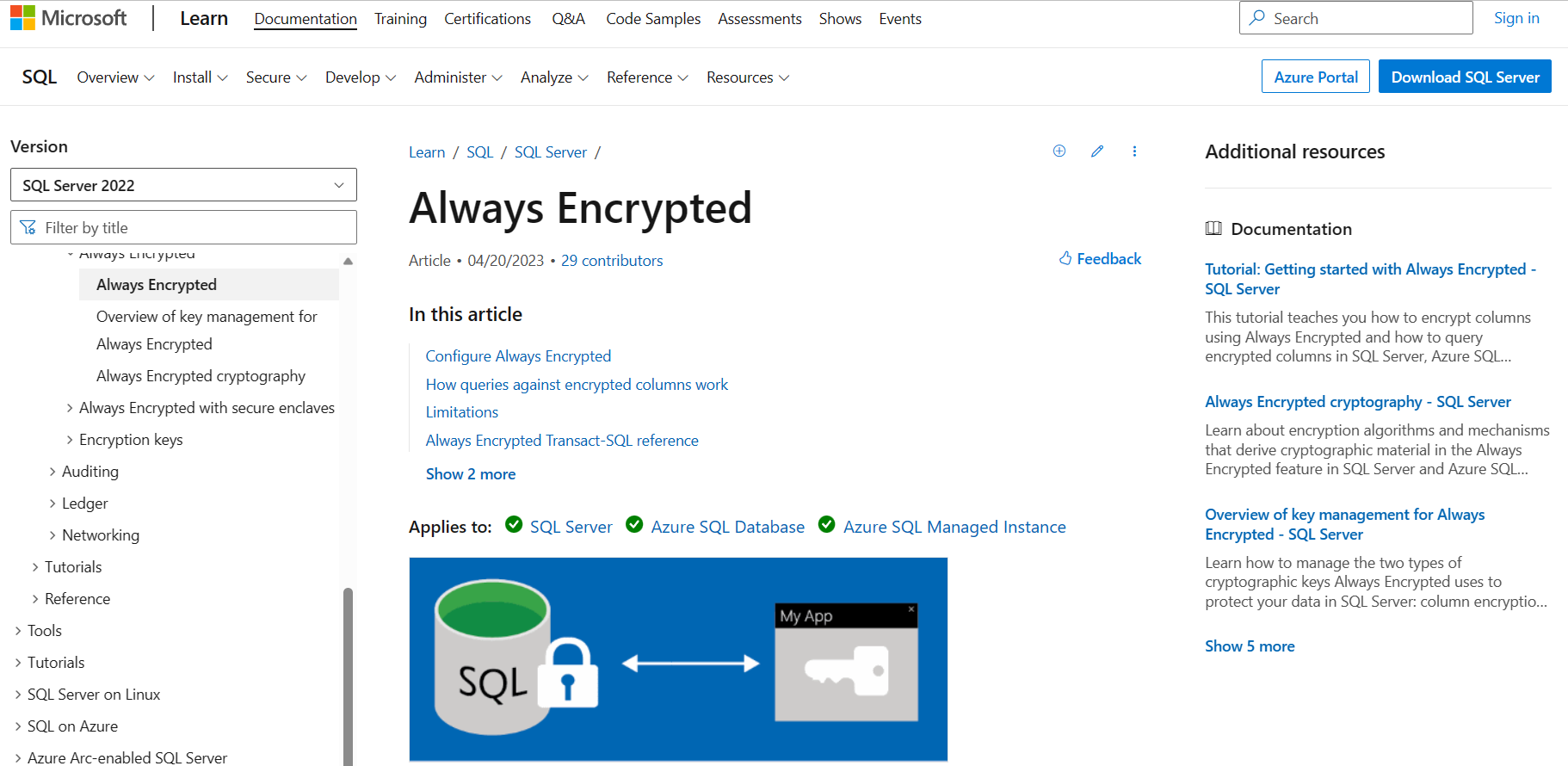
Azure Secure SQL Database Always Encrypted is a feature of Microsoft Azure SQL Database that provides another security-specific layer for sensitive data. Moreover, it protects data at rest and in transit, ensuring that even database administrators or other privileged users cannot access the plaintext values of the encrypted data.
Key Features
1. Client-Side Encryption
Always Encrypted enables client applications to encrypt sensitive data before sending it to the database. As a result, the data remains encrypted throughout its lifecycle and can be decrypted only by an authorized client application.
2. Column-Level Encryption
Always Encrypted allows you to selectively encrypt individual columns in a database table rather than encrypting the entire database. It gives organizations fine-grained control over which data needs encryption, allowing you to balance security and performance requirements.
3. Transparent Data Encryption
The database server stores the encrypted data using a unique encryption format, ensuring the data remains protected even if the database is compromised. The server is unaware of the data values and cannot decrypt them.
Best Practices
The organization needs to plan and manage encryption keys carefully. This is because encryption keys are at the heart of Always Encrypted. Consider the following best practices.
- Use a Secure and Centralized Key Management System - Store encryption keys in a safe and centralized location, separate from the database. Azure Key Vault is a recommended option for managing keys securely.
- Implement Key Rotation and Backup - Regularly rotate encryption keys to mitigate the risks of key compromise. Moreover, establish a key backup strategy to recover encrypted data due to a lost or inaccessible key.
- Control Access to Encryption Keys - Ensure that only authorized individuals or applications have access to the encryption keys. Applying the principle of least privilege and robust access control will prevent unauthorized access to keys.
Azure Key Vault

Azure Key Vault is a cloud service provided by Microsoft Azure that helps safeguard cryptographic keys, secrets, and sensitive information. It is a centralized storage and management system for keys, certificates, passwords, connection strings, and other confidential information required by applications and services. It allows developers and administrators to securely store and tightly control access to their application secrets without exposing them directly in their code or configuration files.
Key Features
1. Key Management
Key Vault provides a secure key management system that allows you to create, import, and manage cryptographic keys for encryption, decryption, signing, and verification.
2. Secret Management
It enables you to securely store (as plain text or encrypted value) and manage secrets such as passwords, API keys, connection strings, and other sensitive information.
3. Certificate Management
Key Vault supports the storage and management of X.509 certificates, allowing you to securely store, manage, and retrieve credentials for application use.
4. Access Control
Key Vault provides fine-grained access control to manage who can perform operations on stored keys and secrets. It integrates with Azure Active Directory (Azure AD) for authentication and authorization.
Best Practices
- Centralized Secrets Management - Consolidate all your application secrets and sensitive information in Key Vault rather than scattering them across different systems or configurations. The benefit of this is it simplifies management and reduces the risk of accidental exposure.
- Use RBAC and Access Policies - Implement role-based access control (RBAC) and define granular access policies to power who can perform operations on Key Vault resources. Follow the principle of least privilege, granting only the necessary permissions to users or applications.
- Secure Key Vault Access - Restrict access to Key Vault resources to trusted networks or virtual networks using virtual network service or private endpoints because it helps prevent unauthorized access to the internet.
Azure AD Multi-Factor Authentication

It is a security feature provided by Microsoft Azure that adds an extra layer of protection to user sign-ins and helps safeguard against unauthorized access to resources. Users must give additional authentication factors beyond just a username and password.
Key Features
1. Multiple Authentication Methods
Azure AD MFA supports a range of authentication methods, including phone calls, text messages (SMS), mobile app notifications, mobile app verification codes, email, and third-party authentication apps. This flexibility allows organizations to choose the methods that best suit their users' needs and security requirements.
2. Conditional Access Policies
Azure AD MFA can configure conditional access policies, allowing organizations to define specific conditions under which MFA (is required), once applied to an organization, on the user location, device trust, application sensitivity, and risk level. This granular control helps organizations strike a balance between security and user convenience.
Best Practices
- Enable MFA for All Users - Implement a company-wide policy to enforce MFA for all users, regardless of their roles or privileges, because it will ensure consistent and comprehensive security across the organization.
- Use Risk-Based Policies - Leverage Azure AD Identity Protection and its risk-based policies to dynamically adjust the level of authentication required based on the perceived risk of each sign-in attempt because it will help balance security and user experience by applying MFA only when necessary.
- Implement Multi-Factor Authentication for Privileged Accounts - Ensure that all privileged accounts, such as administrators and IT staff, are protected with MFA. These accounts have elevated access rights and are prime targets for attackers. Enforcing MFA adds an extra layer of protection to prevent unauthorized access.
Conclusion
In this post, we have introduced the importance of cybersecurity in the cloud space due to dependence on cloud providers. After that we discussed some layers of security in Azure to gain insights about its landscape and see some tools and features available. Of course we can’t ignore the features such as Azure Active Directory Identity Protection, Azure Firewall, Azure Resource Locks, Azure Secure SQL Database Always Encrypted, Azure Key Vault and Azure AD Multi-Factor Authentication by giving an overview on each, its key features and the best practices we can apply to our organization.
Ready to go beyond native Azure tools?
While Azure provides powerful built-in security features, securing sensitive data across multi-cloud environments requires deeper visibility and control.
Request a demo with Sentra to see how our platform complements Azure by discovering, classifying, and protecting sensitive data - automatically and continuously.
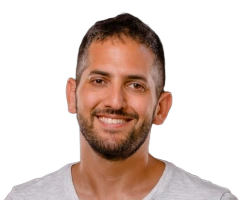
Cloud Vulnerability Management Best Practices for 2025
Cloud Vulnerability Management Best Practices for 2025
What is Cloud Vulnerability Management?
Cloud vulnerability management is a proactive approach to identifying and mitigating security vulnerabilities within your cloud infrastructure, enhancing cloud data security. It involves the systematic assessment of cloud resources and applications to pinpoint potential weaknesses that cybercriminals might exploit. By addressing these vulnerabilities, you reduce the risk of data breaches, service interruptions, and other security incidents that could have a significant impact on your organization.
Common Vulnerabilities in Cloud Security
Before diving into the details of cloud vulnerability management, it's essential to understand the types of vulnerabilities that can affect your cloud environment. Here are some common vulnerabilities that private cloud security experts encounter:
Vulnerable APIs
Application Programming Interfaces (APIs) are the backbone of many cloud services. They allow applications to communicate and interact with the cloud infrastructure. However, if not adequately secured, APIs can be an entry point for cyberattacks. Insecure API endpoints, insufficient authentication, and improper data handling can all lead to vulnerabilities.
# Insecure API endpoint example
import requests
response = requests.get('https://example.com/api/v1/insecure-endpoint')
if response.status_code == 200:
# Handle the response
else:
# Report an error
Misconfigurations
Misconfigurations are one of the leading causes of security breaches in the cloud. These can range from overly permissive access control policies to improperly configured firewall rules. Misconfigurations may leave your data exposed or allow unauthorized access to resources.
# Misconfigured firewall rule
- name: allow-http
sourceRanges:
- 0.0.0.0/0 # Open to the world
allowed:
- IPProtocol: TCP
ports:
- '80'
Data Theft or Loss
Data breaches can result from poor data handling practices, encryption failures, or a lack of proper data access controls. Stolen or compromised data can lead to severe consequences, including financial losses and damage to an organization's reputation.
// Insecure data handling example
import java.io.File;
import java.io.FileReader;
public class InsecureDataHandler {
public String readSensitiveData() {
try {
File file = new File("sensitive-data.txt");
FileReader reader = new FileReader(file);
// Read the sensitive data
reader.close();
} catch (Exception e) {
// Handle errors
}
}
}
Poor Access Management
Inadequate access controls can lead to unauthorized users gaining access to your cloud resources. This vulnerability can result from over-privileged user accounts, ineffective role-based access control (RBAC), or lack of multi-factor authentication (MFA).
# Overprivileged user account
- members:
- user:johndoe@example.com
role: roles/editor
Non-Compliance
Non-compliance with regulatory standards and industry best practices can lead to vulnerabilities. Failing to meet specific security requirements can result in fines, legal actions, and a damaged reputation.
Non-compliance with GDPR regulations can lead to severe financial penalties and legal consequences.
Understanding these vulnerabilities is crucial for effective cloud vulnerability management. Once you can recognize these weaknesses, you can take steps to mitigate them.
Cloud Vulnerability Assessment and Mitigation
Now that you're familiar with common cloud vulnerabilities, it's essential to know how to mitigate them effectively. Mitigation involves a combination of proactive measures to reduce the risk and the potential impact of security issues. Here are some steps to consider:
- Regular Vulnerability Scanning: Implement a robust vulnerability scanning process that identifies and assesses vulnerabilities within your cloud environment. Use automated tools that can detect misconfigurations, outdated software, and other potential weaknesses.
- Access Control: Implement strong access controls to ensure that only authorized users have access to your cloud resources. Enforce the principle of least privilege, providing users with the minimum level of access necessary to perform their tasks.
- Configuration Management: Regularly review and update your cloud configurations to ensure they align with security best practices. Tools like Infrastructure as Code (IaC) and Configuration Management Databases (CMDBs) can help maintain consistency and security.
- Patch Management: Keep your cloud infrastructure up to date by applying patches and updates promptly. Vulnerabilities in the underlying infrastructure can be exploited by attackers, so staying current is crucial.
- Encryption: Use encryption to protect data both at rest and in transit. Ensure that sensitive information is adequately encrypted, and use strong encryption protocols and algorithms.
- Monitoring and Incident Response: Implement comprehensive monitoring and incident response capabilities to detect and respond to security incidents in real time. Early detection can minimize the impact of a breach.
- Security Awareness Training: Train your team on security best practices and educate them about potential risks and how to identify and report security incidents.
Key Features of Cloud Vulnerability Management
Effective cloud vulnerability management provides several key benefits that are essential for securing your cloud environment. Let's explore these features in more detail:
Better Security
Cloud vulnerability management ensures that your cloud environment is continuously monitored for vulnerabilities. By identifying and addressing these weaknesses, you reduce the attack surface and lower the risk of data breaches or other security incidents. This proactive approach to security is essential in an ever-evolving threat landscape.
# Code snippet for vulnerability scanning
import security_scanner
# Initialize the scanner
scanner = security_scanner.Scanner()
# Run a vulnerability scan
scan_results = scanner.scan_cloud_resources()
Cost-Effective
By preventing security incidents and data breaches, cloud vulnerability management helps you avoid potentially significant financial losses and reputational damage. The cost of implementing a vulnerability management system is often far less than the potential costs associated with a security breach.
# Code snippet for cost analysis
def calculate_potential_cost_of_breach():
# Estimate the cost of a data breach
return potential_cost
potential_cost = calculate_potential_cost_of_breach()
if potential_cost > cost_of vulnerability management:
print("Investing in vulnerability management is cost-effective.")
else:
print("The cost of vulnerability management is justified by potential savings.")
Highly Preventative
Vulnerability management is a proactive and preventive security measure. By addressing vulnerabilities before they can be exploited, you reduce the likelihood of a security incident occurring. This preventative approach is far more effective than reactive measures.
# Code snippet for proactive security
import preventive_security_module
# Enable proactive security measures
preventive_security_module.enable_proactive_measures()
Time-Saving
Cloud vulnerability management automates many aspects of the security process. This automation reduces the time required for routine security tasks, such as vulnerability scanning and reporting. As a result, your security team can focus on more strategic and complex security challenges.
# Code snippet for automated vulnerability scanning
import automated_vulnerability_scanner
# Configure automated scanning schedule
automated_vulnerability_scanner.schedule_daily_scan()
Steps in Implementing Cloud Vulnerability Management
Implementing cloud vulnerability management is a systematic process that involves several key steps. Let's break down these steps for a better understanding:
Identification of Issues
The first step in implementing cloud vulnerability management is identifying potential vulnerabilities within your cloud environment. This involves conducting regular vulnerability scans to discover security weaknesses.
# Code snippet for identifying vulnerabilities
import vulnerability_identifier
# Run a vulnerability scan to identify issues
vulnerabilities = vulnerability_identifier.scan_cloud_resources()
Risk Assessment
After identifying vulnerabilities, you need to assess their risk. Not all vulnerabilities are equally critical. Risk assessment helps prioritize which vulnerabilities to address first based on their potential impact and likelihood of exploitation.
# Code snippet for risk assessment
import risk_assessment
# Assess the risk of identified vulnerabilities
priority_vulnerabilities = risk_assessment.assess_risk(vulnerabilities)
Vulnerabilities Remediation
Remediation involves taking action to fix or mitigate the identified vulnerabilities. This step may include applying patches, reconfiguring cloud resources, or implementing access controls to reduce the attack surface.
# Code snippet for vulnerabilities remediation
import remediation_tool
# Remediate identified vulnerabilities
remediation_tool.remediate_vulnerabilities(priority_vulnerabilities)
Vulnerability Assessment Report
Documenting the entire vulnerability management process is crucial for compliance and transparency. Create a vulnerability assessment report that details the findings, risk assessments, and remediation efforts.
# Code snippet for generating a vulnerability assessment report
import report_generator
# Generate a vulnerability assessment report
report_generator.generate_report(priority_vulnerabilities)
Re-Scanning
The final step is to re-scan your cloud environment periodically. New vulnerabilities may emerge, and existing vulnerabilities may reappear. Regular re-scanning ensures that your cloud environment remains secure over time.
# Code snippet for periodic re-scanning
import re_scanner
# Schedule regular re-scans of your cloud resources
re_scanner.schedule_periodic_rescans()
By following these steps, you establish a robust cloud vulnerability management program that helps secure your cloud environment effectively.
Challenges with Cloud Vulnerability Management
While cloud vulnerability management offers many advantages, it also comes with its own set of challenges. Some of the common challenges include:
Challenge | Description |
---|---|
Scalability | As your cloud environment grows, managing and monitoring vulnerabilities across all resources can become challenging. |
Complexity | Cloud environments can be complex, with numerous interconnected services and resources. Understanding the intricacies of these environments is essential for effective vulnerability management. |
Patch Management | Keeping cloud resources up to date with the latest security patches can be a time-consuming task, especially in a dynamic cloud environment. |
Compliance | Ensuring compliance with industry standards and regulations can be challenging, as cloud environments often require tailored configurations to meet specific compliance requirements. |
Alert Fatigue | With a constant stream of alerts and notifications from vulnerability scanning tools, security teams can experience alert fatigue, potentially missing critical security issues. |
Cloud Vulnerability Management Best Practices
To overcome the challenges and maximize the benefits of cloud vulnerability management, consider these best practices:
- Automation: Implement automated vulnerability scanning and remediation processes to save time and reduce the risk of human error.
- Regular Training: Keep your security team well-trained and updated on the latest cloud security best practices.
- Scalability: Choose a vulnerability management solution that can scale with your cloud environment.
- Prioritization: Use risk assessments to prioritize the remediation of vulnerabilities effectively.
- Documentation: Maintain thorough records of your vulnerability management efforts, including assessment reports and remediation actions.
- Collaboration: Foster collaboration between your security team and cloud administrators to ensure effective vulnerability management.
- Compliance Check: Regularly verify your cloud environment's compliance with relevant standards and regulations.
Tools to Help Manage Cloud Vulnerabilities
To assist you in your cloud vulnerability management efforts, there are several tools available. These tools offer features for vulnerability scanning, risk assessment, and remediation. Here are some popular options:
Sentra: Sentra is a cloud-based data security platform that provides visibility, assessment, and remediation for data security. It can be used to discover and classify sensitive data, analyze data security controls, and automate alerts in cloud data stores, IaaS, PaaS, and production environments.
Tenable Nessus: A widely-used vulnerability scanner that provides comprehensive vulnerability assessment and prioritization.
Qualys Vulnerability Management: Offers vulnerability scanning, risk assessment, and compliance management for cloud environments.
AWS Config: Amazon Web Services (AWS) provides AWS Config, as well as other AWS cloud security tools, to help you assess, audit, and evaluate the configurations of your AWS resources.
Azure Security Center: Microsoft Azure's Security Center offers Azure Security tools for continuous monitoring, threat detection, and vulnerability assessment.
Google Cloud Security Scanner: A tool specifically designed for Google Cloud Platform that scans your applications for vulnerabilities.
OpenVAS: An open-source vulnerability scanner that can be used to assess the security of your cloud infrastructure.
Choosing the right tool depends on your specific cloud environment, needs, and budget. Be sure to evaluate the features and capabilities of each tool to find the one that best fits your requirements.
Conclusion
In an era of increasing cyber threats and data breaches, cloud vulnerability management is a vital practice to secure your cloud environment. By understanding common cloud vulnerabilities, implementing effective mitigation strategies, and following best practices, you can significantly reduce the risk of security incidents. Embracing automation and utilizing the right tools can streamline the vulnerability management process, making it a manageable and cost-effective endeavor. Remember that security is an ongoing effort, and regular vulnerability scanning, risk assessment, and remediation are crucial for maintaining the integrity and safety of your cloud infrastructure. With a robust cloud vulnerability management program in place, you can confidently leverage the benefits of the cloud while keeping your data and assets secure.
If you want to learn more about how you can implement a robust cloud vulnerability management program to confidently harness the power of the cloud while keeping your data and assets secure, request a demo today.
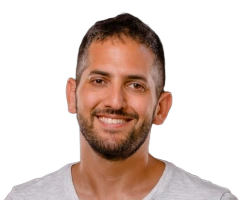
Data Leakage Detection for AWS Bedrock
Data Leakage Detection for AWS Bedrock
Amazon Bedrock is a fully managed service that streamlines access to top-tier foundation models (FMs) from premier AI startups and Amazon, all through a single API. This service empowers users to leverage cutting-edge generative AI technologies by offering a diverse selection of high-performance FMs from innovators like AI21 Labs, Anthropic, Cohere, Meta, Mistral AI, Stability AI, and Amazon itself. Amazon Bedrock allows for seamless experimentation and customization of these models to fit specific needs, employing techniques such as fine-tuning and Retrieval Augmented Generation (RAG).
Additionally, it supports the development of agents capable of performing tasks with enterprise systems and data sources. As a serverless offering, it removes the complexities of infrastructure management, ensuring secure and easy deployment of generative AI features within applications using familiar AWS services, all while maintaining robust security, privacy, and responsible AI standards.
Why Are Enterprises Using AWS Bedrock
Enterprises are increasingly using AWS Bedrock for several key reasons:
- Diverse Model Selection: Offers access to a curated selection of high-performing foundation models (FMs) from both leading AI startups and Amazon itself, providing a comprehensive range of options to suit various use cases and preferences. This diversity allows enterprises to select the most suitable models for their specific needs, whether they require language generation, image processing, or other AI capabilities.
- Streamlined Integration: Simplifies the process of adopting and integrating generative AI technologies into existing systems and applications. With its unified API and serverless architecture, enterprises can seamlessly incorporate these advanced AI capabilities without the need for extensive infrastructure management or specialized expertise. This streamlines the development and deployment process, enabling faster time-to-market for AI-powered solutions.
- Customization Capabilities: Facilitates experimentation and customization, allowing enterprises to fine-tune and adapt the selected models to better align with their unique requirements and data environments. Techniques such as fine-tuning and Retrieval Augmented Generation (RAG) enable enterprises to refine the performance and accuracy of the models, ensuring optimal results for their specific use cases.
- Security and Compliance Focus: Prioritizes security, privacy, and responsible AI practices, providing enterprises with the confidence that their data and AI deployments are protected and compliant with regulatory standards. By leveraging AWS's robust security infrastructure and compliance measures, enterprises can deploy generative AI applications with peace of mind.
AWS Bedrock Data Privacy & Security Concerns
The rise of AI technologies, while promising transformative and major benefits, also introduces significant security risks. As enterprises increasingly integrate AI into their operations, like with AWS Bedrock, they face challenges related to data privacy, model integrity, and ethical use. AI systems, particularly those involving generative models, can be susceptible to adversarial attacks, unintended data extraction, and unintended biases, which can lead to compromised data security and regulatory violations.
Training Data Concerns
Training data is the backbone of machine learning and artificial intelligence systems. The quality, diversity, and integrity of this data are critical for building robust models. However, there are significant risks associated with inadvertently using sensitive data in training datasets, as well as the unintended retrieval and leakage of such data.
These risks can have severe consequences, including breaches of privacy, legal repercussions, and erosion of public trust.
Accidental Usage of Sensitive Data in Training Sets
Inadvertently including sensitive data in training datasets can occur for various reasons, such as insufficient data vetting, poor anonymization practices, or errors in data aggregation. Sensitive data may encompass personally identifiable information (PII), financial records, health information, intellectual property, and more.
The consequences of training models on such data are multifaceted:
- Data Privacy Violations: When models are trained on sensitive data, they might inadvertently learn and reproduce patterns that reveal private information. This can lead to direct privacy breaches if the model outputs or intermediate states expose this data.
- Regulatory Non-Compliance: Many jurisdictions have stringent regulations regarding the handling and processing of sensitive data, such as GDPR in the EU, HIPAA in the US, and others. Accidental inclusion of sensitive data in training sets can result in non-compliance, leading to heavy fines and legal actions.
- Bias and Ethical Concerns: Sensitive data, if not properly anonymized or aggregated, can introduce biases into the model. For instance, using demographic data can inadvertently lead to models that discriminate against certain groups.
These risks require strong security measures and responsible AI practices to protect sensitive information and comply with industry standards. AWS Bedrock provides a ready solution to power foundation models and Sentra provides a complementary solution to ensure compliance and integrity of data these models use and output. Let’s explore how this combination and each component delivers its respective capility.
Prompt Response Monitoring With Sentra
Sentra can detect sensitive data leakage in near real-time by scanning and classifying all prompt responses generated by AWS Bedrock, by analyzing them using Sentra’s Data Detection and Response (DDR) security module.
Data exfiltration might occur if AWS Bedrock prompt responses are used to return data outside of an organization - for example using a chatbot interface connected directly to a user facing application.
By analyzing the prompt responses, Sentra can ensure that both sensitive data acquired through fine-tuning models and data retrieved using Retrieval-Augmented Generation (RAG) methods are protected. This protection is effective within minutes of any data exfiltration attempt.
To activate the detection module, there are 3 prerequisites:
- The customer should enable AWS Bedrock Model Invocation Logging to an S3 destination(instructions here) in the customer environment.
- A new Sentra tenant for the customer should be created/set up.
- The customer should install the Sentra copy Lambda using Sentra’s Cloudformation template for its DDR module (documentation provided by Sentra).
Once the prerequisites are fulfilled, Sentra will automatically analyze the prompt responses and will be able to provide real-time security threat alerts based on the defined set of policies configured for the customer at Sentra.
Here is the full flow which describes how Sentra scans the prompts in near real-time:
- Sentra’s setup involves using AWS Lambda to handle new files uploaded to the Sentra S3 bucket configured in customer cloud, which logs all responses from AWS Bedrock prompts. When a new file arrives, our Lambda function copies it into Sentra’s prompt response buckets.
- Next, another S3 trigger kicks off enrichment of each response with extra details needed for detecting sensitive information.
- Our real-time data classification engine then gets to work, sorting the data from the responses into categories like emails, phone numbers, names, addresses, and credit card info. It also identifies the context, such as intellectual property or customer data.
- Finally, Sentra uses this classified information to spot any sensitive data. We then generate an alert and notify our customers, also sending the alert to any relevant downstream systems.
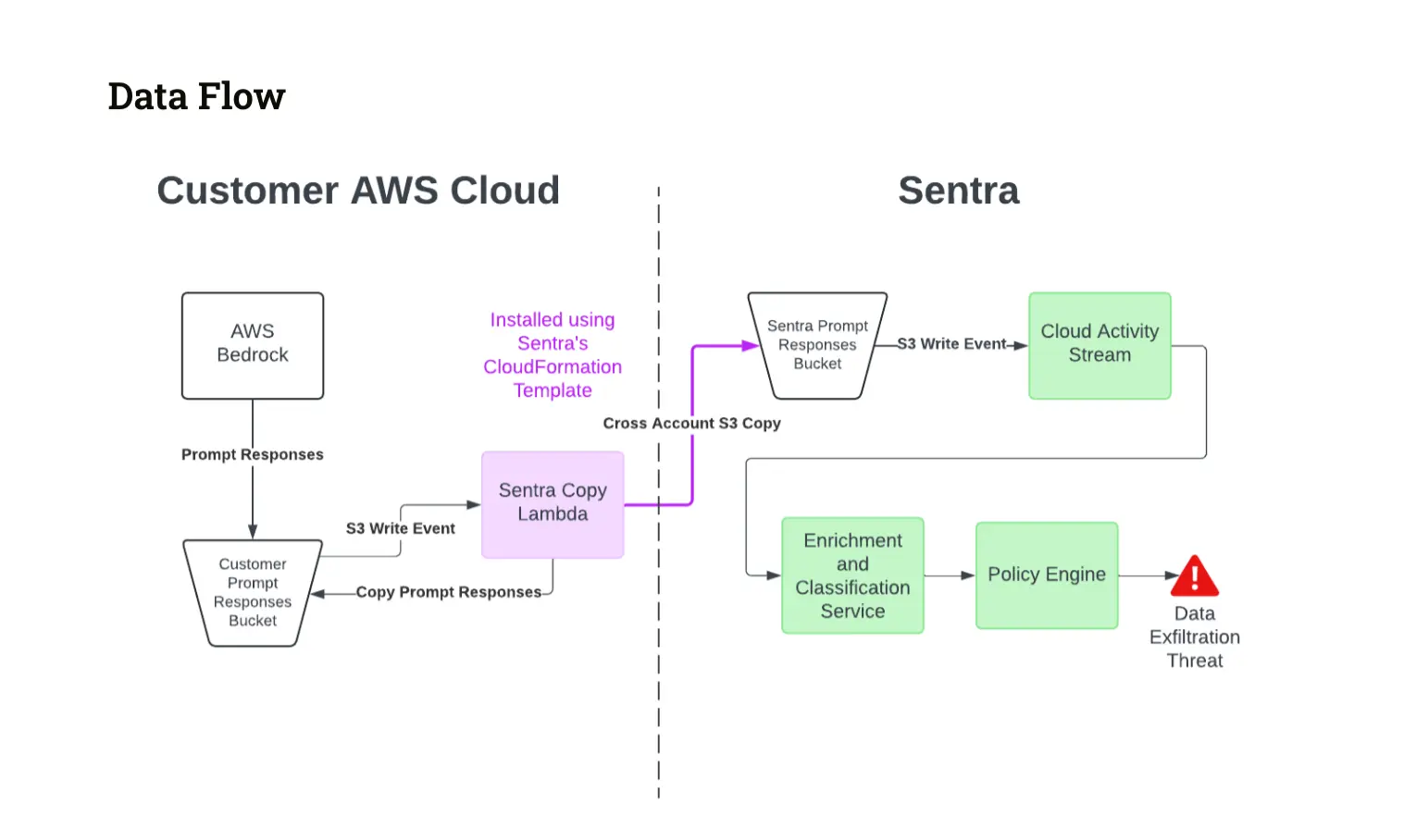
Sentra can push these alerts downstream into 3rd party systems, such as SIEMs, SOARs, ticketing systems, and messaging systems (Slack, Teams, etc.).
Sentra’s data classification engine provides three methods of classification:
- Regular expressions
- List classifiers
- AI models
Further, Sentra allows the customer to add its own classifiers for their own business-specific needs, apart from the 150+ data classifiers which Sentra provides out of the box.
Sentra’s sensitive data detection also provides control for setting a threshold of the amount of sensitive data exfiltrated through Bedrock over time (similar to a rate limit) to reduce the rate of false positives for non-critical exfiltration events.
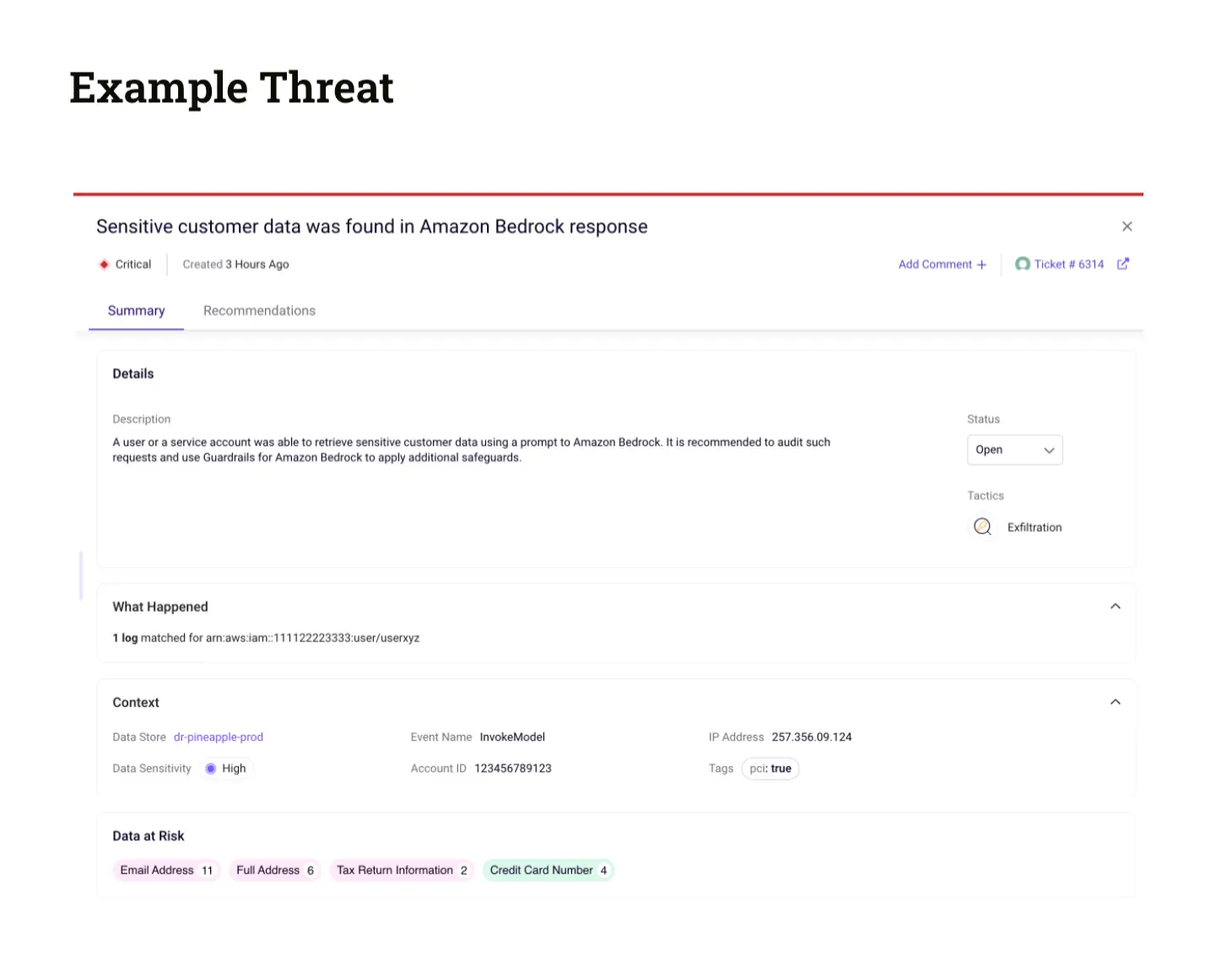
Conclusion
There is a pressing push for AI integration and automation to enable businesses to improve agility, meet growing cloud service and application demands, and improve user experiences - but to do so while simultaneously minimizing risks. Early warning to potential sensitive data leakage or breach is critical to achieving this goal.
Sentra's data security platform can be used in the entire development pipeline to classify, test and verify that models do not leak sensitive information, serving the developers, but also helping them to increase confidence among their buyers. By adopting Sentra, organizations gain the ability to build out automation for business responsiveness and improved experiences, with the confidence knowing their most important asset — their data — will remain secure.
If you want to learn more, request a live demo with our data security experts.
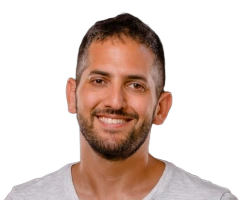
What is Sensitive Data Exposure and How to Prevent It
What is Sensitive Data Exposure and How to Prevent It
What is Sensitive Data Exposure?
Sensitive data exposure occurs when security measures fail to protect sensitive information from external and internal threats. This leads to unauthorized disclosure of private and confidential data. Attackers often target personal data, such as financial information and healthcare records, as it is valuable and exploitable.
Security teams play a critical role in mitigating sensitive data exposures. They do this by implementing robust security measures. This includes eliminating malicious software, enforcing strong encryption standards, and enhancing access controls. Yet, even with the most sophisticated security measures in place, data breaches can still occur. They often happen through the weakest links in the system.
Organizations must focus on proactive measures to prevent data exposures. They should also put in place responsive strategies to effectively address breaches. By combining proactive and responsive measures, as stated below, organizations can protect sensitive data exposure. They can also maintain the trust of their customers.
Proactive Measures | Responsive Strategies |
---|---|
Implementation of appropriate security posture controls for sensitive data, such as encryption, data masking, de-identification, etc. | Security audits with patch management ensure the masking of affected data to minimize the attack surface and eradicate threats. |
Sensitive data access restrictions through least privilege principles enforcement. | Promptly identifying and reacting through incident response systems with adequate alerting. |
Enablement of comprehensive logging mechanisms to capture and monitor activities on sensitive data. | Investigating the root cause of the breach to prevent similar incidents from occurring in the future. |
Alignment with cyber protection regulations and compliance requirements through adherence to strict cyber policies. | Implementing additional custom security measures to strengthen the overall security posture. |
Difference Between Data Exposure and Data Breach
Both data exposure and data breaches involve unauthorized access or disclosure of sensitive information. However, they differ in their intent and the underlying circumstances.
Data Exposure
Data exposure occurs when sensitive information is inadvertently disclosed or made accessible to unauthorized individuals or entities. This exposure can happen due to various factors. These include misconfigured systems, human error, or inadequate security measures. Data exposure is typically unintentional. The exposed data may not be actively targeted or exploited.
Data Breach
A data breach, on the other hand, is a deliberate act of unauthorized access to sensitive information with the intent to steal, manipulate, or exploit it. Data breaches are often carried out by cybercriminals or malicious actors seeking financial gain, identity theft, or to disrupt an organization's operations.
Key Differences
The table below summarizes the key differences between sensitive data exposure and data breaches:
Features | Data Exposure | Data Breach |
---|---|---|
Intent | Unintentional | Intentional |
Underlying Factor | Human error, misconfigured systems, inadequate security | Deliberate attacks by cybercriminals or malicious actors |
Impact | Can still lead to privacy violations and reputational damage | Often more severe impacts, including fraud and financial losses, identity theft, and disruption of operations |
Solutions | Following security best practices, continuous monitoring and SecOps literacy | Robust security measures with discrete monitoring and alerting for anomaly detection and remediation |
Types of Sensitive Data Exposure
Attackers relentlessly pursue sensitive data. They create increasingly sophisticated and inventive methods to breach security systems and compromise valuable information. Their motives range from financial gain to disruption of operations. Ultimately, this causes harm to individuals and organizations alike. There are three main types of data breaches that can compromise sensitive information:
Availability Breach
An availability breach occurs when authorized users are temporarily or permanently denied access to sensitive data. Ransomware commonly uses this method to extort organizations. Such disruptions can impede business operations and hinder essential services. They can also result in financial losses. Addressing and mitigating these breaches is essential to ensure uninterrupted access and business continuity.
Confidentiality Breach
A confidentiality breach occurs when unauthorized entities access sensitive data, infringing upon its privacy and confidentiality. The consequences can be severe. They can include financial fraud, identity theft, reputational harm, and legal repercussions. It's crucial to maintain strong security measures. Doing so prevents breaches and preserves sensitive information's integrity.
Integrity Breach
An integrity breach occurs when unauthorized individuals or entities alter or modify sensitive data. AI LLM training is particularly vulnerable to this breach form. This compromises the data's accuracy and reliability. This manipulation of data can result in misinformation, financial losses, and diminished trust in data quality. Vigilant measures are essential to protect data integrity. They also help reduce the impact of breaches.
How Sensitive Data Gets Exposed
Sensitive data, including vital information like Personally Identifiable Information (PII), financial records, and healthcare data, forms the backbone of contemporary organizations. Unfortunately, weak encryption, unreliable application programming interfaces, and insufficient security practices from development and security teams can jeopardize this invaluable data. Such lapses lead to critical vulnerabilities, exposing sensitive data at three crucial points:
Data in Transit
Data in transit refers to the transfer of data between locations, such as from a user's device to a server or between servers. This data is a prime target for attackers due to its often unencrypted state, making it vulnerable to interception. Key factors contributing to data exposure in transit include weak encryption, insecure protocols, and the risk of man-in-the-middle attacks. It is crucial to address these vulnerabilities to enhance the security of data during transit.
Data at Rest
While data at rest is less susceptible to interception than data in transit, it remains vulnerable to attacks. Enterprises commonly face internal exposure to sensitive data when they have misconfigurations or insufficient access controls on data at rest. Oversharing and insufficient access restrictions heighten the risk in data lakes and warehouses that house Personally Identifiable Information (PII). To mitigate this risk, it is important to implement robust access controls and monitoring measures. This ensures restricted access and vigilant tracking of data access patterns.
Data in Use
Data in use is the most vulnerable to attack, as it is often unencrypted and can be accessed by multiple users and applications. When working in cloud computing environments, dev teams usually gather the data and cache it within the mounts or in-memory to boost performance and reduce I/O. Such data causes sensitive data exposure vulnerabilities as other teams or cloud providers can access the data. The security teams need to adopt standard data handling practices. For example, they should clean the data from third-party or cloud mounts after use and disable caching.
What Causes Sensitive Data Exposure?
Sensitive data exposure results from a combination of internal and external factors. Internally, DevSecOps and Business Analytics teams play a significant role in unintentional data exposures. External threats usually come from hackers and malicious actors. Mitigating these risks requires a comprehensive approach to safeguarding data integrity and maintaining a resilient security posture.
Internal Causes of Sensitive Data Exposure
- No or Weak Encryption: Encryption and decryption algorithms are the keys to safeguarding data. Sensitive data exposures occur due to weak cryptography protocols. They also occur due to a lack of encryption or hashing mechanisms.
- Insecure Passwords: Insecure password practices and insufficient validation checks compromise enterprise security, facilitating data exposure.
- Unsecured Web Pages: JSON payloads get delivered from web servers to frontend API handlers. Attackers can easily exploit the data transaction between the server and client when users browse unsecure web pages with weak SSL and TLS certificates.
- Poor Access Controls and Misconfigurations: Insufficient multi-factor authentication (MFA) or excessive permissioning and unreliable security posture management contribute to sensitive data exposure through misconfigurations.
- Insider Threat Attacks: Current or former employees may unintentionally or intentionally target data, posing risks to organizational security and integrity.
External Causes of Sensitive Data Exposure
- SQL Injection: SQL Injection happens when attackers introduce malicious queries and SQL blocks into server requests. This lets them tamper with backend queries to retrieve or alter data, causing SQL injection attacks.
- Network Compromise: A network compromise occurs when unauthorized users gain control of backend services or servers. This compromises network integrity, risking resource theft or data alteration.
- Phishing Attacks: Phishing attacks contain malicious links. They exploit urgency, tricking recipients into disclosing sensitive information like login credentials or personal details.
- Supply Chain Attacks: When compromised, Third-party service providers or vendors exploit the dependent systems and unintentionally expose sensitive data publicly.
Impact of Sensitive Data Exposure
Exposing sensitive data poses significant risks. It encompasses private details like health records, user credentials, and biometric data. Accountability, governed by acts like the Accountability Act, mandates organizations to safeguard granular user information. Failure to prevent unauthorized exposure can result in severe consequences. This can include identity theft and compromised user privacy. It can also lead to regulatory and legal repercussions and potential corruption of databases and infrastructure. Organizations must focus on stringent measures to mitigate these risks.
.jpeg)
Examples of Sensitive Data Exposure
Prominent companies, including Atlassian, LinkedIn, and Dubsmash, have unfortunately become notable examples of sensitive data exposure incidents. Analyzing these cases provides insights into the causes and repercussions of such data exposure. It offers valuable lessons for enhancing data security measures.
Atlassian Jira (2019)
In 2019, Atlassian Jira, a project management tool, experienced significant data exposure. The exposure resulted from a configuration error. A misconfiguration in global permission settings allowed unauthorized access to sensitive information. This included names, email addresses, project details, and assignee data. The issue originated from incorrect permissions granted during the setup of filters and dashboards in JIRA.
LinkedIn (2021)
LinkedIn, a widely used professional social media platform, experienced a data breach where approximately 92% of user data was extracted through web scraping. The security incident was attributed to insufficient webpage protection and the absence of effective mechanisms to prevent web crawling activity.
Equifax (2017)
In 2017, Equifax Ltd., the UK affiliate of credit reporting company Equifax Inc., faced a significant data breach. Hackers infiltrated Equifax servers in the US, impacting over 147 million individuals, including 13.8 million UK users. Equifax failed to meet security obligations. It outsourced security management to its US parent company. This led to the exposure of sensitive data such as names, addresses, phone numbers, dates of birth, Equifax membership login credentials, and partial credit card information.
Cost of Compliance Fines
Data exposure poses significant risks, whether at rest or in transit. Attackers target various dimensions of sensitive information. This includes protected health data, biometrics for AI systems, and personally identifiable information (PII). Compliance costs are subject to multiple factors influenced by shifting regulatory landscapes. This is true regardless of the stage.
Enterprises failing to safeguard data face substantial monetary fines or imprisonment. The penalty depends on the impact of the exposure. Fines can range from millions to billions, and compliance costs involve valuable resources and time. Thus, safeguarding sensitive data is imperative for mitigating reputation loss and upholding industry standards.
How to Determine if You Are Vulnerable to Sensitive Data Exposure?
Detecting security vulnerabilities in the vast array of threats to sensitive data is a challenging task. Unauthorized access often occurs due to lax data classification and insufficient access controls. Enterprises must adopt additional measures to assess their vulnerability to data exposure.
Deep scans, validating access levels, and implementing robust monitoring are crucial steps. Detecting unusual access patterns is crucial. In addition, using advanced reporting systems to swiftly detect anomalies and take preventive measures in case of a breach is an effective strategy. It proactively safeguards sensitive data.
Automation is key as well - to allow burdened security teams the ability to keep pace with dynamic cloud use and data proliferation. Automating discovery and classification, freeing up resources, and doing so in a highly autonomous manner without requiring huge setup and configuration efforts can greatly help.
How to Prevent Sensitive Data Exposure
Effectively managing sensitive data demands rigorous preventive measures to avert exposure. Widely embraced as best practices, these measures serve as a strategic shield against breaches. The following points focus on specific areas of vulnerability. They offer practical solutions to either eliminate potential sensitive data exposures or promptly respond to them:
Assess Risks Associated with Data
The initial stages of data and access onboarding serve as gateways to potential exposure. Conducting a thorough assessment, continual change monitoring, and implementing stringent access controls for critical assets significantly reduces the risks of sensitive data exposure. This proactive approach marks the first step to achieving a strong data security posture.
Minimize Data Surface Area
Overprovisioning and excessive sharing create complexities. This turns issue isolation, monitoring, and maintenance into challenges. Without strong security controls, every part of the environment, platform, resources, and data transactions poses security risks. Opting for a less-is-more approach is ideal. This is particularly true when dealing with sensitive information like protected health data and user credentials. By minimizing your data attack surface, you mitigate the risk of cloud data leaks.
Store Passwords Using Salted Hashing Functions and Leverage MFA
Securing databases, portals, and services hinges on safeguarding passwords. This prevents unauthorized access to sensitive data. It is crucial to handle password protection and storage with precision. Use advanced hashing algorithms for encryption and decryption. Adding an extra layer of security through multi-factor authentication strengthens the defense against potential breaches even more.
Disable Autocomplete and Caching
Cached data poses significant vulnerabilities and risks of data breaches. Enterprises often use auto-complete features, requiring the storage of data on local devices for convenient access. Common instances include passwords stored in browser sessions and cache. In cloud environments, attackers exploit computing instances. They access sensitive cloud data by exploiting instances where data caching occurs. Mitigating these risks involves disabling caching and auto-complete features in applications. This effectively prevents potential security threats.
Fast and Effective Breach Response
Instances of personal data exposure stemming from threats like man-in-the-middle and SQL injection attacks necessitate swift and decisive action. External data exposure carries a heightened impact compared to internal incidents. Combatting data breaches demands a responsive approach. It's often facilitated by widely adopted strategies. These include Data Detection and Response (DDR), Security Orchestration, Automation, and Response (SOAR), User and Entity Behavior Analytics (UEBA), and the renowned Zero Trust Architecture featuring Predictive Analytics (ZTPA).
Tools to Prevent Sensitive Data Exposure
Shielding sensitive information demands a dual approach—internally and externally. Unauthorized access can be prevented through vigilant monitoring, diligent analysis, and swift notifications to both security teams and affected users. Effective tools, whether in-house or third-party, are indispensable in preventing data exposure.
Data Security Posture Management (DSPM) is designed to meet the changing requirements of security, ensuring a thorough and meticulous approach to protecting sensitive data. Tools compliant with DSPM standards usually feature data tokenization and masking, seamlessly integrated into their services. This ensures that data transmission and sharing remains secure.
These tools also often have advanced security features. Examples include detailed access controls, specific access patterns, behavioral analysis, and comprehensive logging and monitoring systems. These features are essential for identifying and providing immediate alerts about any unusual activities or anomalies.
Sentra emerges as an optimal solution, boasting sophisticated data discovery and classification capabilities. It continuously evaluates data security controls and issues automated notifications. This addresses critical data vulnerabilities ingrained in its core.
Conclusion
In the era of cloud transformation and digital adoption, data emerges as the driving force behind innovations. Personal Identifiable Information (PII), which is a specific type of sensitive data, is crucial for organizations to deliver personalized offerings that cater to user preferences. The value inherent in data, both monetarily and personally, places it at the forefront, and attackers continually seek opportunities to exploit enterprise missteps.
Failure to adopt secure access and standard security controls by data-holding enterprises can lead to sensitive data exposure. Unaddressed, this vulnerability becomes a breeding ground for data breaches and system compromises. Elevating enterprise security involves implementing data security posture management and deploying robust security controls. Advanced tools with built-in data discovery and classification capabilities are essential to this success. Stringent security protocols fortify the tools, safeguarding data against vulnerabilities and ensuring the resilience of business operations.
If you want to learn more about how you can prevent sensitive data exposure, request a demo with our data security experts today.
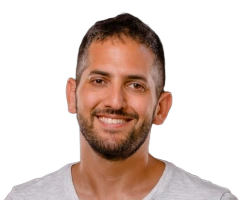
AWS Security Groups: Best Practices, EC2, & More
AWS Security Groups: Best Practices, EC2, & More
What are AWS Security Groups?
AWS Security Groups are a vital component of AWS's network security and cloud data security. They act as a virtual firewall that controls inbound and outbound traffic to and from AWS resources. Each AWS resource, such as Amazon Elastic Compute Cloud (EC2) instances or Relational Database Service (RDS) instances, can be associated with one or more security groups.
Security groups operate at the instance level, meaning that they define rules that specify what traffic is allowed to reach the associated resources. These rules can be applied to both incoming and outgoing traffic, providing a granular way to manage access to your AWS resources.
How Do AWS Security Groups Work?
To comprehend how AWS Security Groups, in conjunction with AWS security tools, function within the AWS ecosystem, envision them as gatekeepers for inbound and outbound network traffic. These gatekeepers rely on a predefined set of rules to determine whether traffic is permitted or denied.
Here's a simplified breakdown of the process:
Inbound Traffic: When an incoming packet arrives at an AWS resource, AWS evaluates the rules defined in the associated security group. If the packet matches any of the rules allowing the traffic, it is permitted; otherwise, it is denied.
Outbound Traffic: Outbound traffic from an AWS resource is also controlled by the security group's rules. It follows the same principle: traffic is allowed or denied based on the rules defined for outbound traffic.

Security groups are stateful, which means that if you allow inbound traffic from a specific IP address, the corresponding outbound response traffic is automatically allowed. This simplifies rule management and ensures that related traffic is not blocked.
Types of Security Groups in AWS
There are two types of AWS Security Groups:
Types of AWS Security Groups | Description |
---|---|
EC2-Classic Security Groups | These are used with instances launched in the EC2-Classic network. It is an older network model, and AWS encourages the use of Virtual Private Cloud (VPC) for new instances. |
VPC Security Groups | These are used with instances launched within a Virtual Private Cloud (VPC). VPCs offer more advanced networking features and are the standard for creating isolated network environments in AWS. |
For this guide, we will focus on VPC Security Groups as they are more versatile and widely used.
How to Use Multiple Security Groups in AWS
In AWS, you can associate multiple security groups with a single resource. When multiple security groups are associated with an instance, AWS combines their rules. This is done in a way that allows for flexibility and ease of management. The rules are evaluated as follows:
- Union: Rules from different security groups are merged. If any security group allows the traffic, it is permitted.
- Deny Overrides Allow: If a rule in one security group denies the traffic, it takes precedence over any rule that allows the traffic in another security group.
- Default Deny: If a packet doesn't match any rule, it is denied by default.
Let's explore how to create, manage, and configure security groups in AWS.
Security Groups and Network ACLs
Before diving into security group creation, it's essential to understand the difference between security groups and Network Access Control Lists (NACLs). While both are used to control inbound and outbound traffic, they operate at different levels.
Security Groups: These operate at the instance level, filtering traffic to and from the resources (e.g., EC2 instances). They are stateful, which means that if you allow incoming traffic from a specific IP, outbound response traffic is automatically allowed.
Network ACLs (NACLs): These operate at the subnet level and act as stateless traffic filters. NACLs define rules for all resources within a subnet, and they do not automatically allow response traffic.
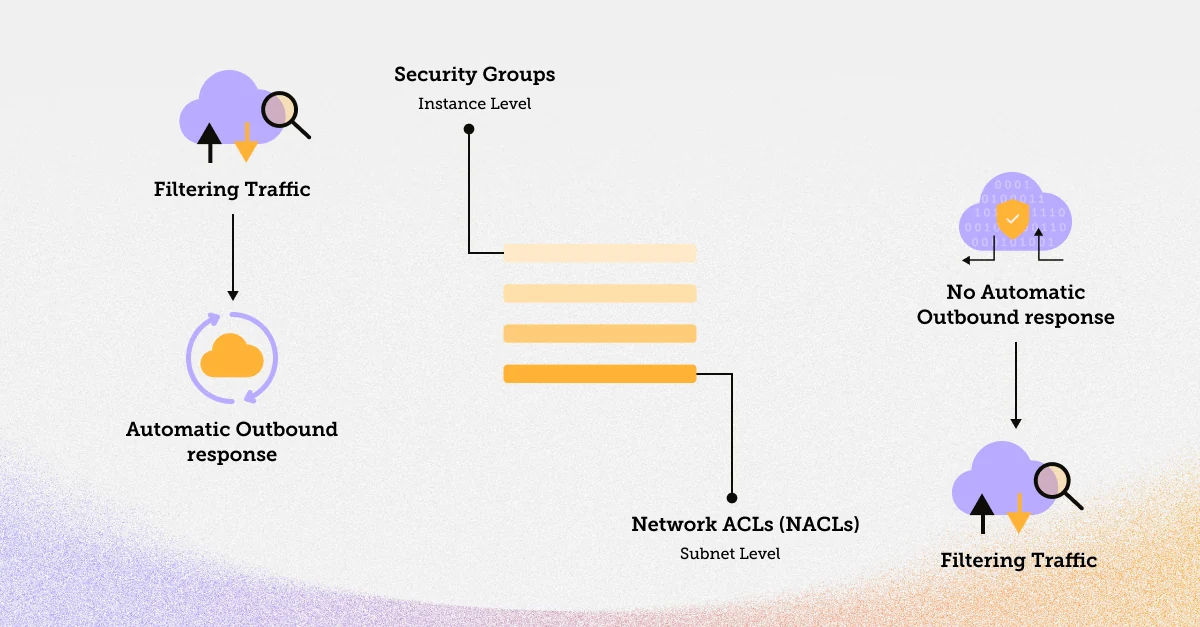
For the most granular control over traffic, use security groups for instance-level security and NACLs for subnet-level security.
AWS Security Groups Outbound Rules
AWS Security Groups are defined by a set of rules that specify which traffic is allowed and which is denied. Each rule consists of the following components:
- Type: The protocol type (e.g., TCP, UDP, ICMP) to which the rule applies.
- Port Range: The range of ports to which the rule applies.
- Source/Destination: The IP range or security group that is allowed to access the resource.
- Allow/Deny: Whether the rule allows or denies traffic that matches the rule criteria.
Now, let's look at how to create a security group in AWS.
Creating a Security Group in AWS
To create a security group in AWS (through the console), follow these steps:
Steps | Description |
---|---|
Sign in to the AWS Management Console | Log in to your AWS account. |
Navigate to the EC2 Dashboard | Select the "EC2" service. |
Access the Security Groups Section | In the EC2 Dashboard, under the "Network & Security" category, click on "Security Groups" in the navigation pane on the left. |
Create a New Security Group | Click the "Create Security Group" button. |
Configure Security Group Settings |
|
Add Outbound Rules | Similarly, add rules for outbound traffic under the "Outbound Rules" section. |
Review and Create | Double-check your rule settings and click "Create Security Group." |
Your security group is now created and ready to be associated with AWS resources.
Below, we'll demonstrate how to create a security group using the AWS CLI.
aws ec2 create-security-group --group-name MySecurityGroup --description
"My Security Group"
In the above command:
--group-name specifies the name of your security group.
--description provides a brief description of the security group.
After executing this command, AWS will return the security group's unique identifier, which is used to reference the security group in subsequent commands.
Adding a Rule to a Security Group
Once your security group is created, you can easily add, edit, or remove rules. To add a new rule to an existing security group through a console, follow these steps:
- Select the security group you want to modify in the EC2 Dashboard.
- In the "Inbound Rules" or "Outbound Rules" tab, click the "Edit Inbound Rules" or "Edit Outbound Rules" button.
- Click the "Add Rule" button.
- Define the rule with the appropriate type, port range, and source/destination.
- Click "Save Rules."
To create a Security Group, you can also use the create-security-group command, specifying a name and description. After creating the Security Group, you can add rules to it using the authorize-security-group-ingress and authorize-security-group-egress commands. The code snippet below adds an inbound rule to allow SSH traffic from a specific IP address range.
# Create a new Security Group
aws ec2 create-security-group --group-name MySecurityGroup --description "My Security Group"
# Add an inbound rule to allow SSH traffic from a specific IP address
aws ec2 authorize-security-group-ingress --group-id sg-0123456789abcdef0 --protocol tcp --port 22 --cidr 203.0.113.0/24
Assigning a Security Group to an EC2 Instance
To secure your EC2 instances using security groups through the console, follow these steps:
- Navigate to the EC2 Dashboard in the AWS Management Console.
- Select the EC2 instance to which you want to assign a security group.
- Click the "Actions" button, choose "Networking," and then click "Change Security Groups."
- In the "Assign Security Groups" dialog, select the desired security group(s) and click "Save."
Your EC2 instance is now associated with the selected security group(s), and its inbound and outbound traffic is governed by the rules defined in those groups.
# Launch an EC2 instance and associate it with a Security Group
aws ec2 run-instances --image-id ami-12345678 --count 1 --instance-type t2.micro --key-name MyKeyPair --security-group-ids sg-0123456789abcdef0
When launching an EC2 instance, you can specify the Security Groups to associate with it. In the example above, we associate the instance with a Security Group using the --security-group-ids flag.
Deleting a Security Group
To delete a security group via the AWS Management Console, follow these steps:
- In the EC2 Dashboard, select the security group you wish to delete.
- Check for associated instances and disassociate them, if necessary.
- Click the "Actions" button, and choose "Delete Security Group."
- Confirm the deletion when prompted.
- Receive confirmation of the security group's removal.
# Delete a Security Group
aws ec2 delete-security-group --group-id sg-0123456789abcdef0
To delete a Security Group, you can use the delete-security-group command and specify the Security Group's ID through AWS CLI.
AWS Security Groups Best Practices
Here are some additional best practices to keep in mind when working with AWS Security Groups:
Enable Tracking and Alerting
One best practice is to enable tracking and alerting for changes made to your Security Groups. AWS provides a feature called AWS Config, which allows you to track changes to your AWS resources, including Security Groups. By setting up AWS Config, you can receive notifications when changes occur, helping you detect and respond to any unauthorized modifications quickly.
Delete Unused Security Groups
Over time, you may end up with unused or redundant Security Groups in your AWS environment. It's essential to regularly review your Security Groups and delete any that are no longer needed. This reduces the complexity of your security policies and minimizes the risk of accidental misconfigurations.
Avoid Incoming Traffic Through 0.0.0.0/0
One common mistake in Security Group configurations is allowing incoming traffic from '0.0.0.0/0,' which essentially opens up your resources to the entire internet. It's best to avoid this practice unless you have a specific use case that requires it. Instead, restrict incoming traffic to only the IP addresses or IP ranges necessary for your applications.
Use Descriptive Rule Names
When creating Security Group rules, provide descriptive names that make it clear why the rule exists. This simplifies rule management and auditing.
Implement Least Privilege
Follow the principle of least privilege by allowing only the minimum required access to your resources. Avoid overly permissive rules.
Regularly Review and Update Rules
Your security requirements may change over time. Regularly review and update your Security Group rules to adapt to evolving security needs.
Avoid Using Security Group Rules as the Only Layer of Defense
Security Groups are a crucial part of your defense, but they should not be your only layer of security. Combine them with other security measures, such as NACLs and web application firewalls, for a comprehensive security strategy.
Leverage AWS Identity and Access Management (IAM)
Use AWS IAM to control access to AWS services and resources. IAM roles and policies can provide fine-grained control over who can modify Security Groups and other AWS resources.
Implement Network Segmentation
Use different Security Groups for different tiers of your application, such as web servers, application servers, and databases. This helps in implementing network segmentation and ensuring that resources only communicate as necessary.
Regularly Audit and Monitor
Set up auditing and monitoring tools to detect and respond to security incidents promptly. AWS provides services like AWS CloudWatch and AWS CloudTrail for this purpose.
Conclusion
Securing your cloud environment is paramount when using AWS, and Security Groups play a vital role in achieving this goal. By understanding how Security Groups work, creating and managing rules, and following best practices, you can enhance the security of your AWS resources. Remember to regularly review and update your security group configurations to adapt to changing security requirements and maintain a robust defense against potential threats. With the right approach to AWS Security Groups, you can confidently embrace the benefits of cloud computing while ensuring the safety and integrity of your applications and data.